DISCOVER DATA INTELLIGENCE
Join 20,000 of your peers in San Francisco, June 9–12, for 700+ sessions, keynotes and training at the world’s largest data, analytics and AI conference
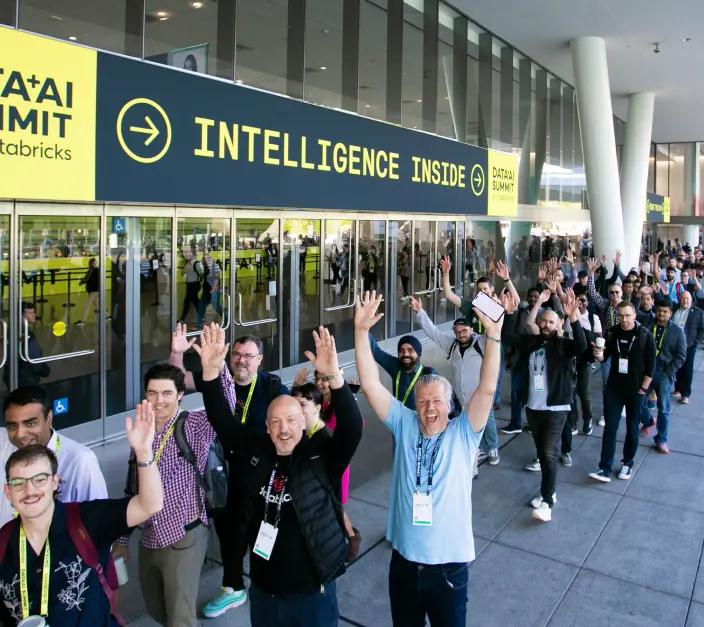
Featured speakers
Data + AI Summit speakers include leading experts, researchers and open source contributors — from Databricks and across the data and AI community
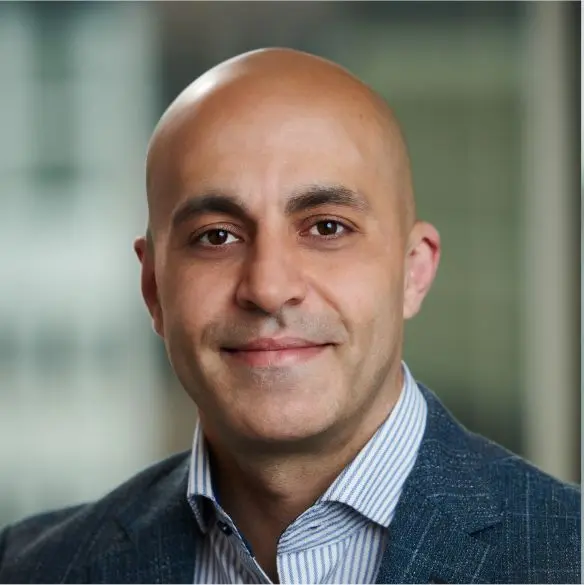
Ali
Ghodsi
Co-founder and CEO,
Databricks

Jamie
Dimon
Chairman and CEO,
JPMorgan Chase
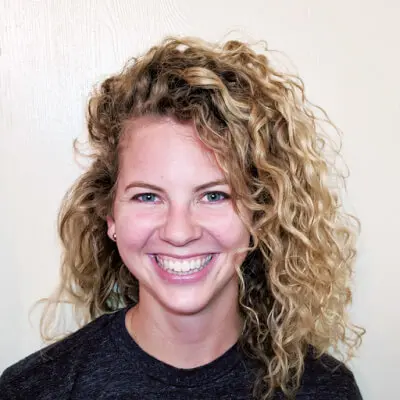
Kasey
Uhlenhuth
Director of Product Management,
Databricks
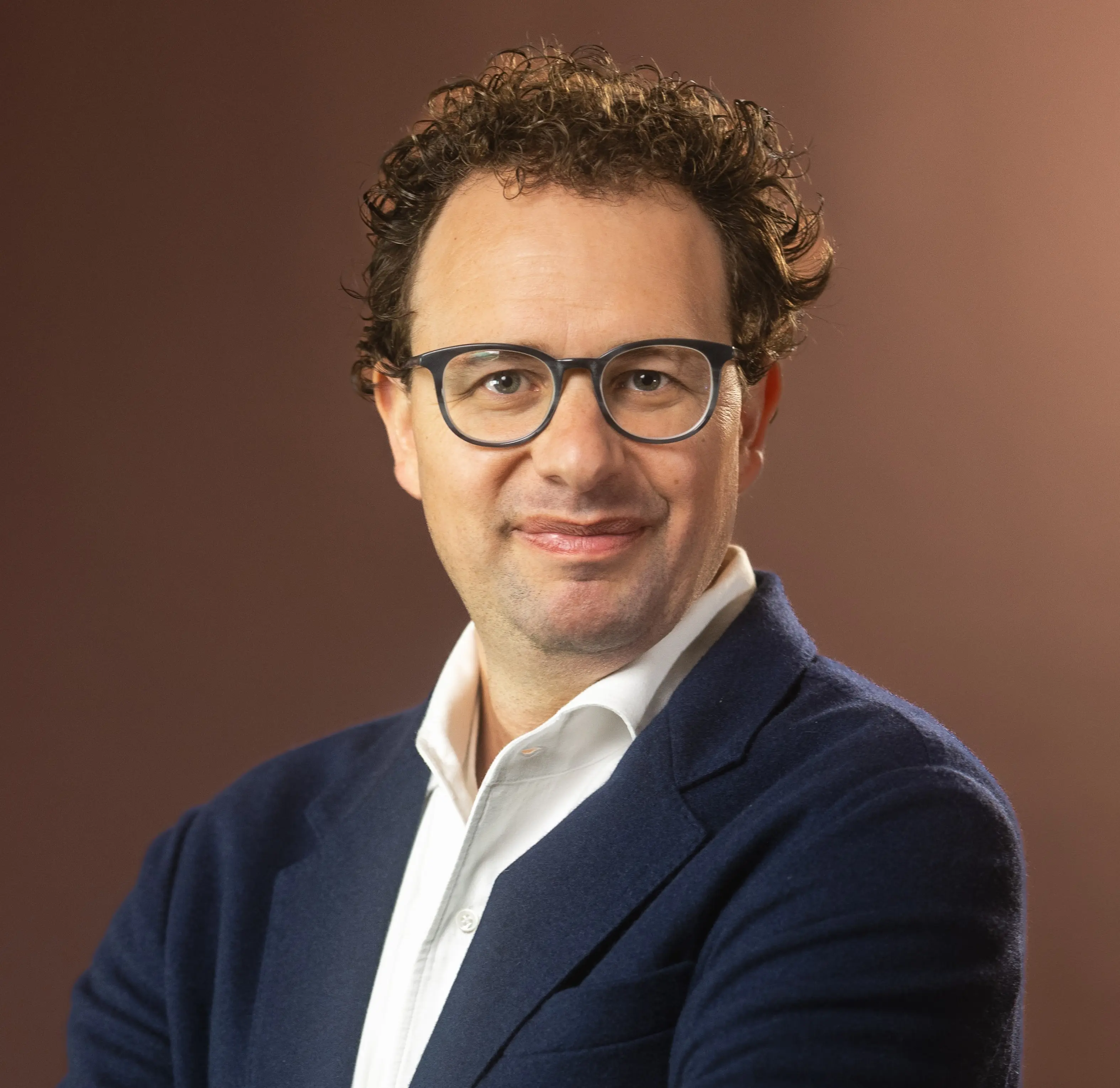
Dario
Amodei
Co-founder and CEO,
Anthropic
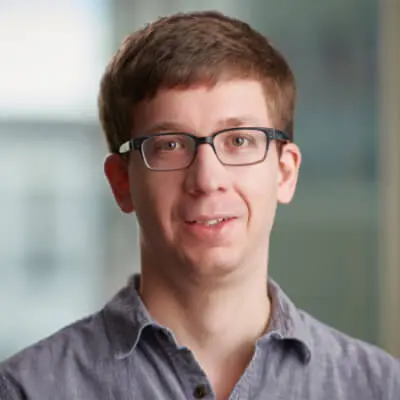
Matei
Zaharia
Original Creator of Apache Spark™ and MLflow; CTO,
Databricks
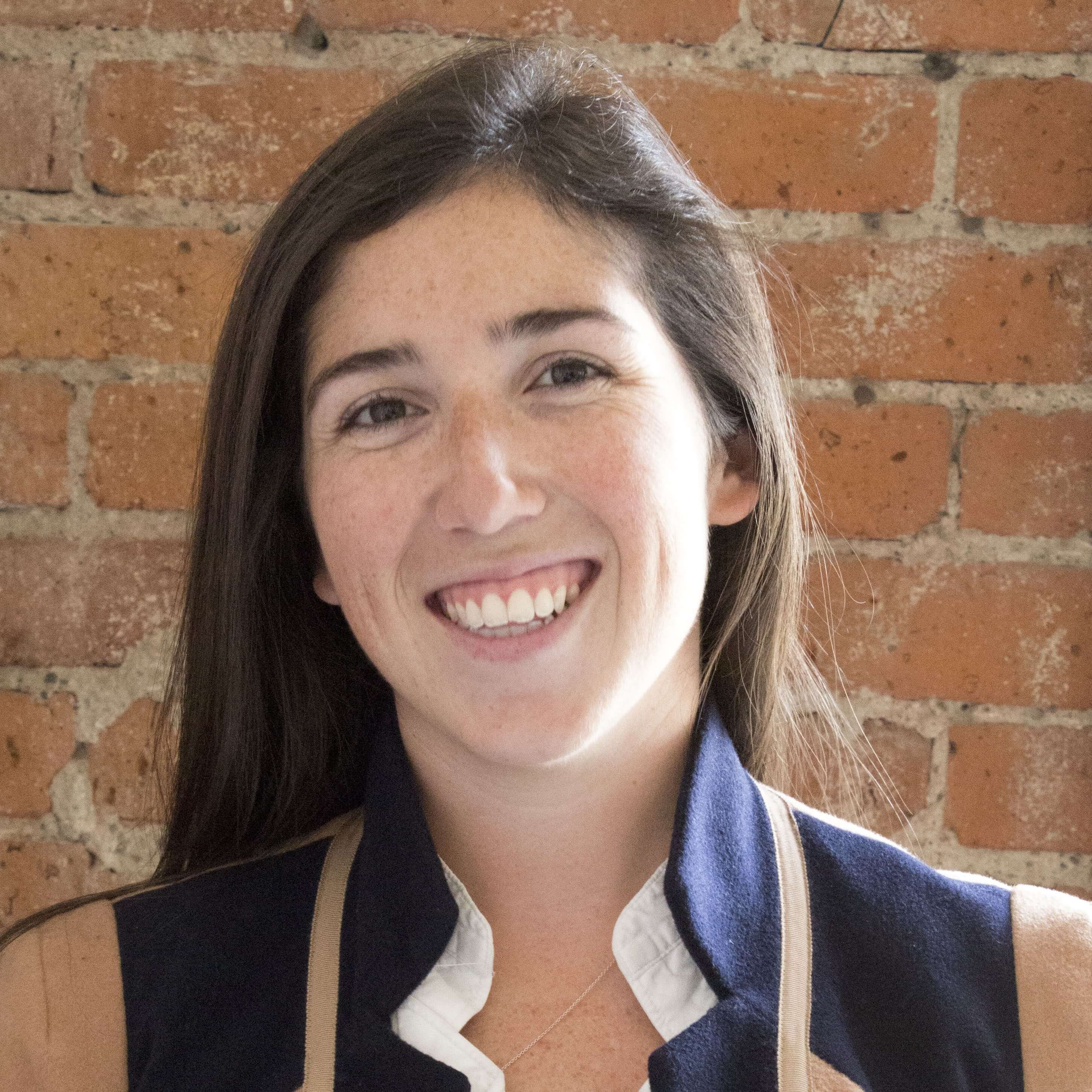
Miranda
Luna
Sr Manager Product Management,
Databricks
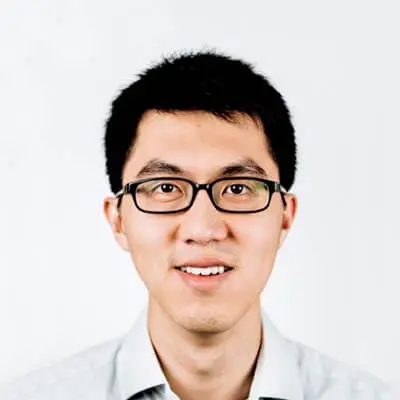
Reynold
Xin
Co-founder and Chief Architect,
Databricks

Sharon
Zhou
CEO & Cofounder,
Lamini
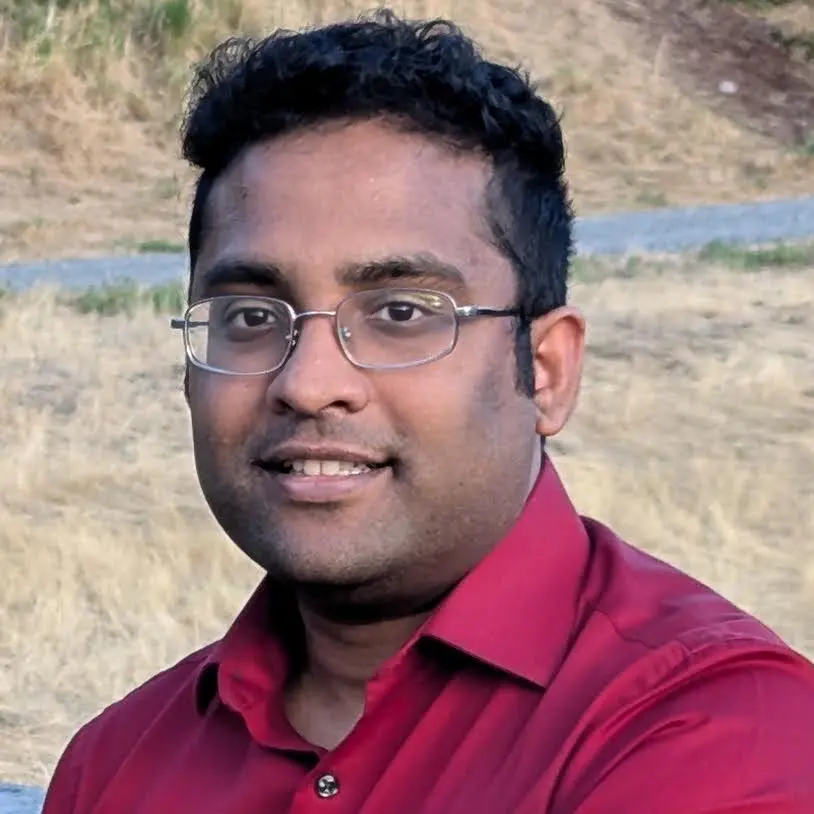
Arvindram
Krishnamoorthy
Principal Architect, ML Solutions,
T-Mobile
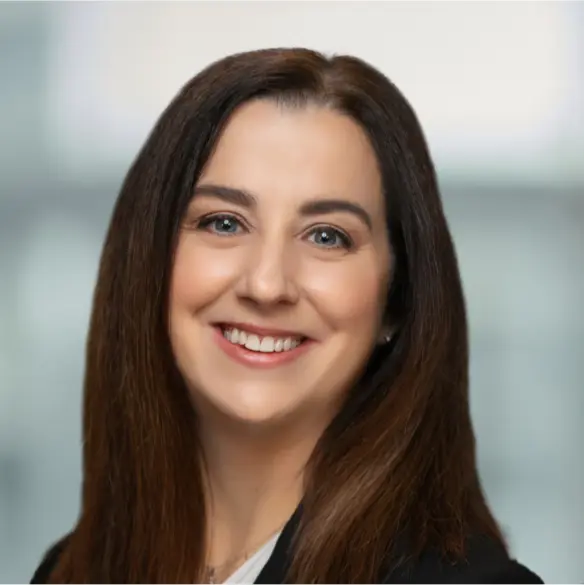
Robin
Sutara
Field CTO,
Databricks
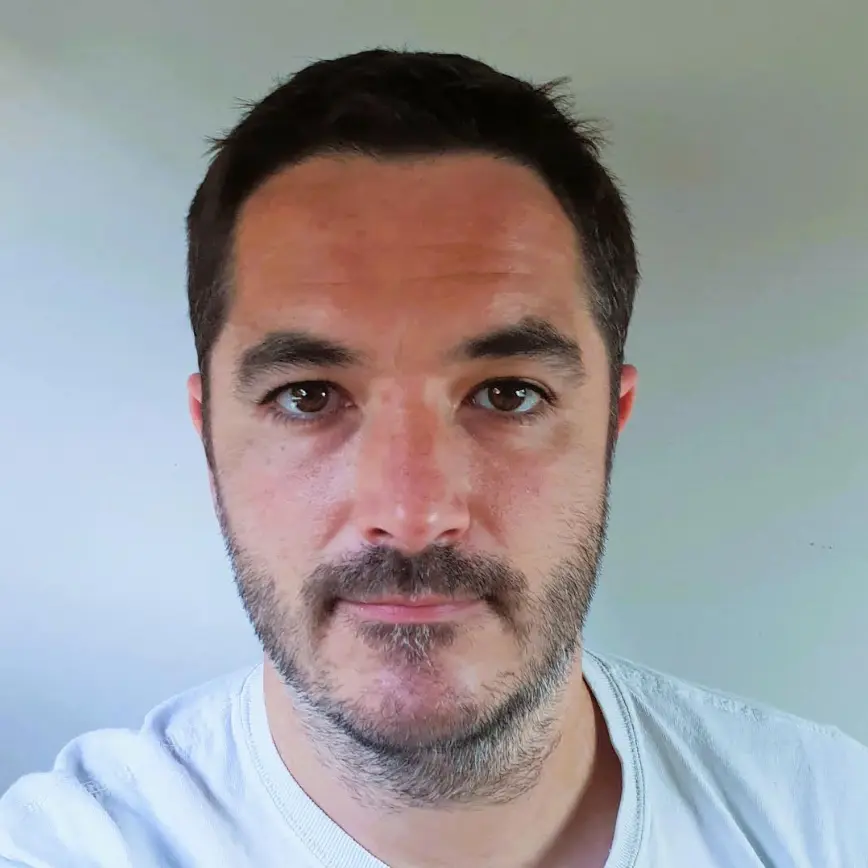
Felix
Baker
Head of Data Services,
SEGA Europe Limited
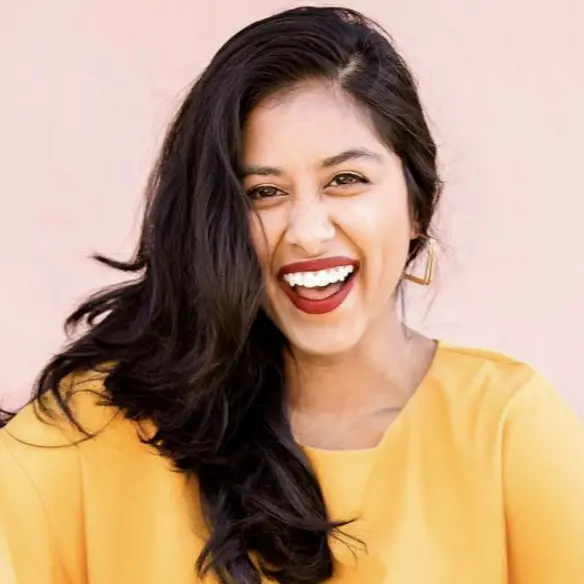
Aparna
Dhinakaran
Co-Founder and Chief Product Officer,
Arize
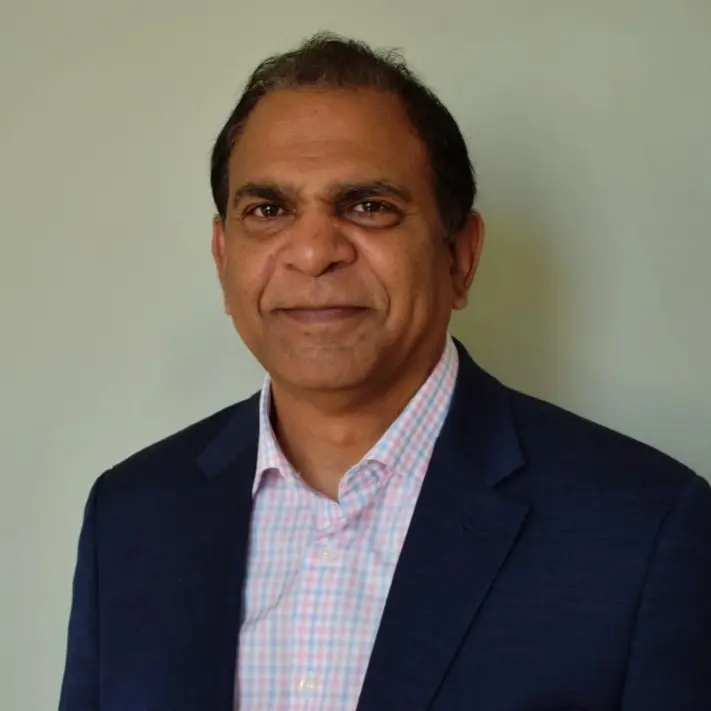
Murali
Vridhachalam
VP & Head of Cloud, Data, and Analytics,
Gilead Sciences
Sessions
700+ sessions — from data intelligence to data warehousing, governance to AI. Here are some key highlights.
Self-Service Assortment and Space Analytics at Walmart Scale
Optimizing EV Charging Experience: Machine Learning for Accurate Charge Time Estimation
Evolving Data Insights With Privacy at Mastercard
Powering Secure and Scalable Data Governance at PepsiCo With Unity Catalog Open APIs
Build Data Pipelines with DLT
Data Management and Governance With UC
Why attend
Join thousands of data leaders, engineers, scientists and architects to explore the convergence of data and AI
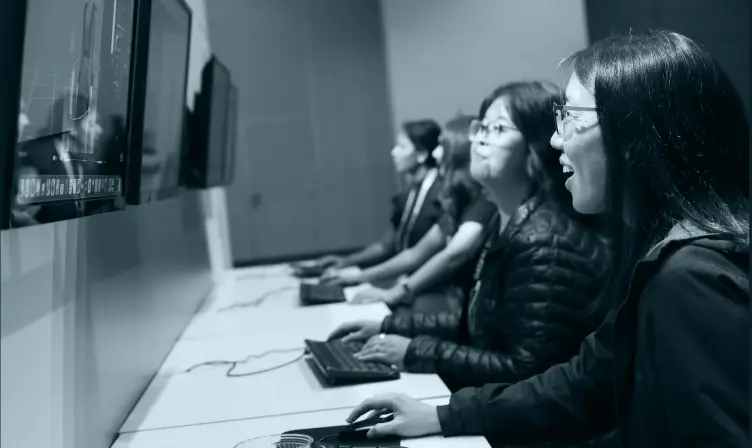
learn
Discover the Latest Technologies
Explore the latest advances in Delta Lake, Apache Iceberg™, agentic systems, MLflow, Apache Spark™, Unity Catalog, DLT, DSPy, LangChain, PyTorch, dbt, Trino, as well as the newest innovations from our sponsors, partners and the Databricks Data Intelligence Platform.
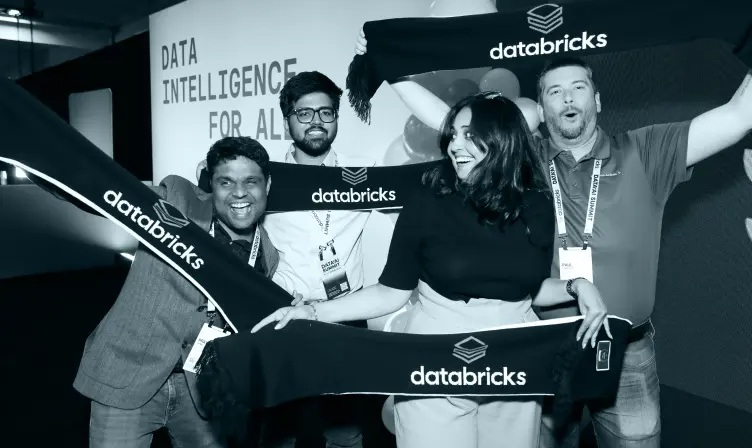
NETWORK
Make Meaningful Connections
Interact with thousands of your peers from the data and AI community, and grow your professional network through social meetups, the Expo and exclusive event parties.
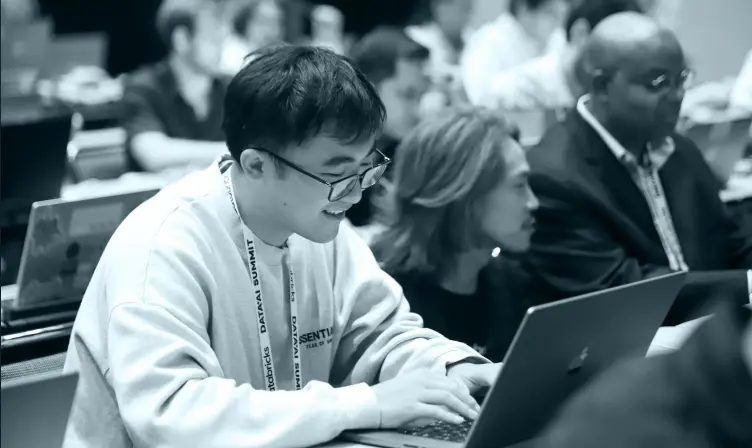
LEVEL UP YOUR SKILLS
Training and Certification
Sharpen your expertise with instructor-led, half-day training. Boost your credentials with our hands-on, lab-based format and get onsite certifications.
Pricing
Reduced prices are available for group purchases and government, military, education and nonprofit attendees
GENERAL ADMISSION
$1,895
Sponsors
Data + AI Summit couldn’t happen without our awesome sponsors. Interested in sponsoring? Reach out to our Sponsorship Management team to learn about available opportunities.