Distributed Deep Learning for Cancer Cell Typing and Tumor Purity
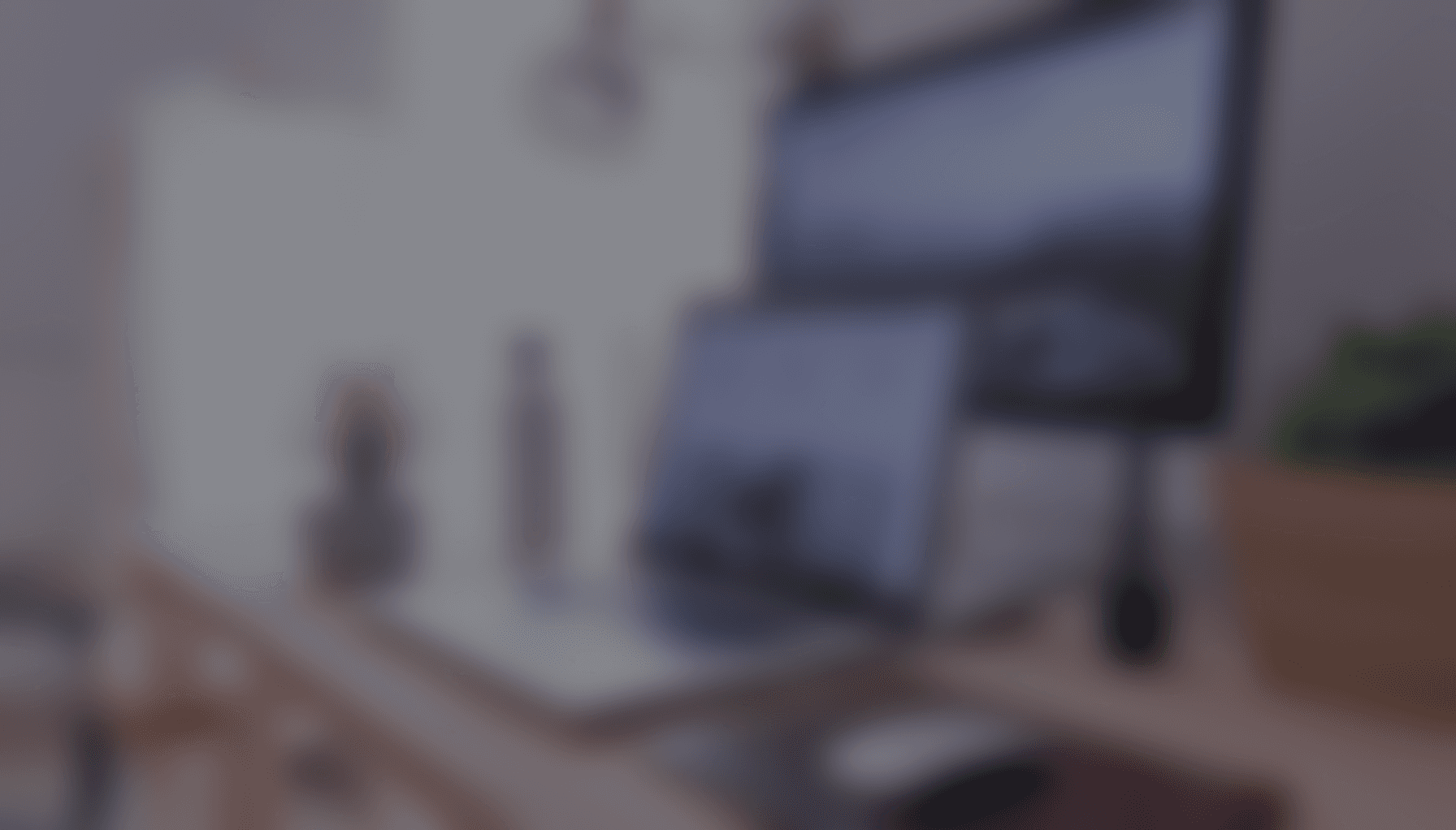
OVERVIEW
EXPERIENCE | In Person |
---|---|
TYPE | Lightning Talk |
TRACK | Data Science and Machine Learning |
INDUSTRY | Health and Life Sciences |
TECHNOLOGIES | AI/Machine Learning, Apache Spark, Delta Lake |
SKILL LEVEL | Advanced |
DURATION | 20 min |
DOWNLOAD SESSION SLIDES |
At Providence St. Joseph Health, we're pioneering digital pathology workflows using an AI/ML vision model for accurate tumor analysis from H&E stained slides. Leveraging Azure Databricks, our innovative approach distributes complex image processing tasks across a Spark cluster, achieving a tenfold speed increase per Whole Slide Image (WSI). Our focus includes overcoming OpenSlide file management challenges through caching across executors, implementing parallel processing with a pre-trained StarDist model for thousands of WSI tiles, and applying GIS-style spatial joins for precise cell labeling. This breakthrough significantly enhances our large-scale genomics research, propelling advancements in digital pathology.
SESSION SPEAKERS
Robert Kramer
/Principal Data Scientist
Providence Health & Services