DATA INTELLIGENCE FOR ALL
JUNE 10–13,2024
MOSCONE CENTER, SAN FRANCISCO
JOIN THE GLOBAL DATA COMMUNITY FOR 500+ SESSIONS AND EXPLORE HOW DATA INTELLIGENCE ENABLES EVERY ORGANIZATION TO HARNESS THE POWER OF GENAI ON THEIR OWN DATA.
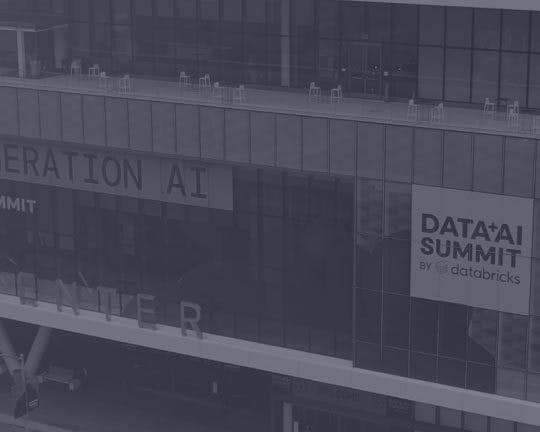
FEATURED SPEAKERS
Join leading experts, researchers and open source contributors — from Databricks and across the data and AI community — who will speak at Data + AI Summit.
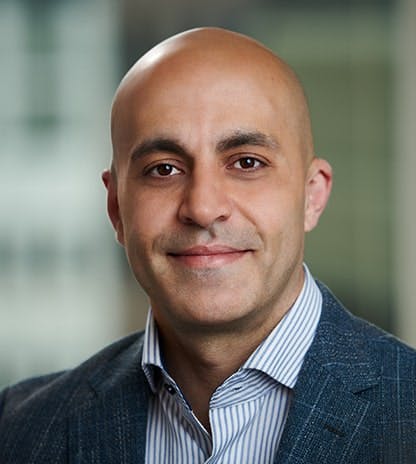
Ali
Ghodsi
Co-founder and CEO,
Databricks
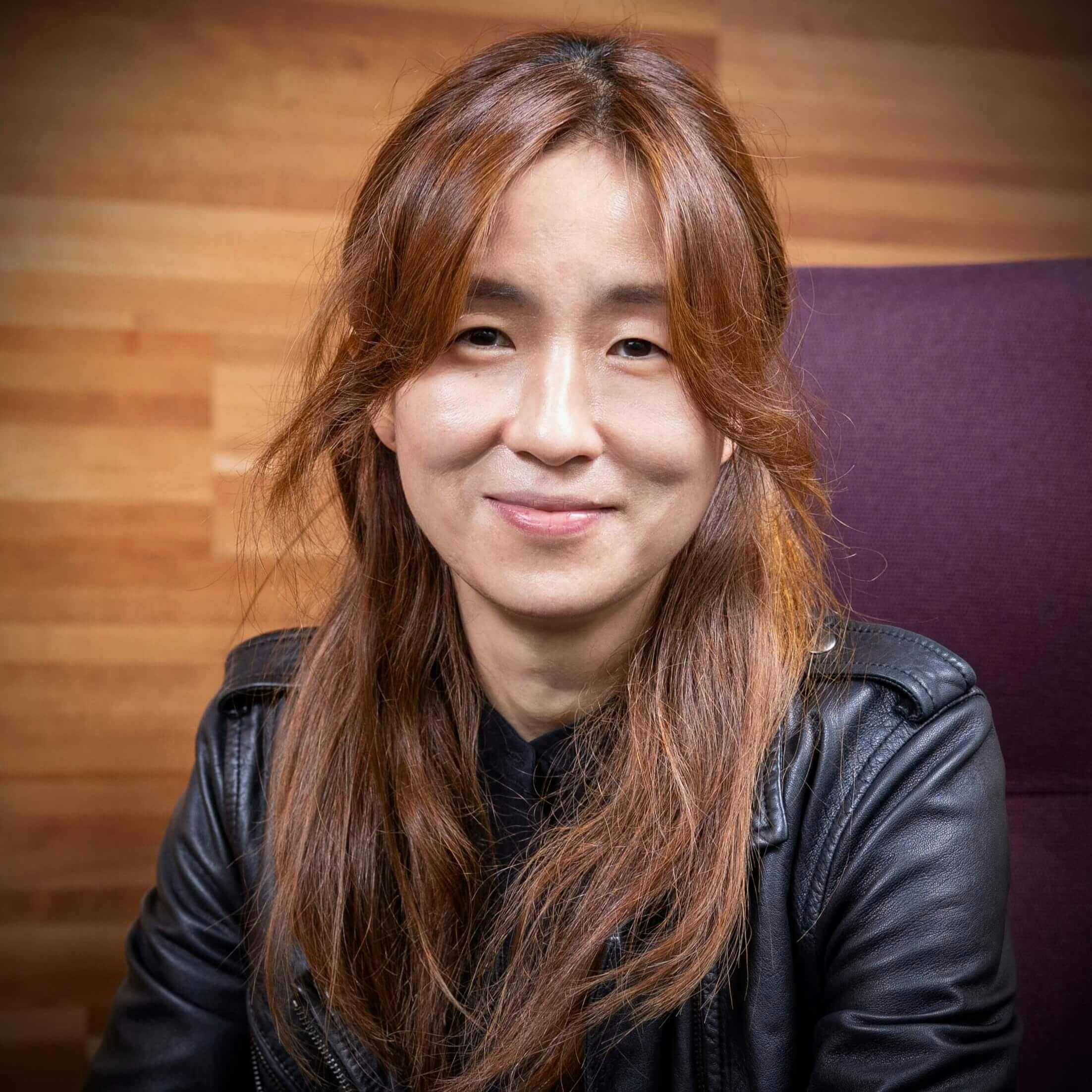
Yejin
Choi
Professor and MacArthur Fellow, University of Washington,
Senior Research Director for Commonsense AI, AI2
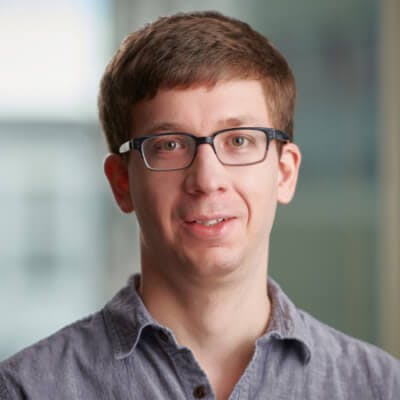
Matei
Zaharia
Original Creator of Apache Spark™ and MLflow; Chief Technologist,
Databricks
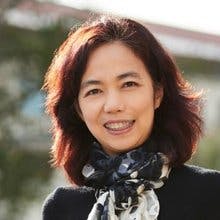
Fei-Fei
Li
Professor, Stanford University and Co-Director,
Stanford Human-Centered AI Institute
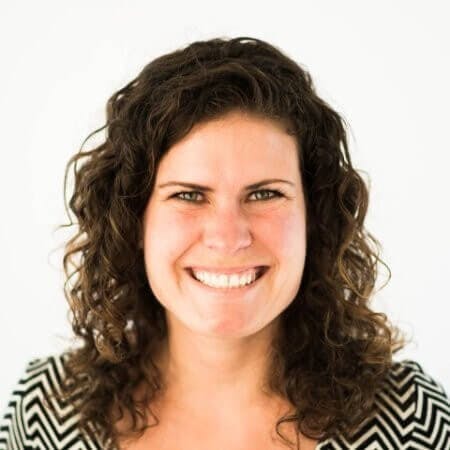
Jackie
Brosamer
Head of AI and Data Platform Engineering,
Block
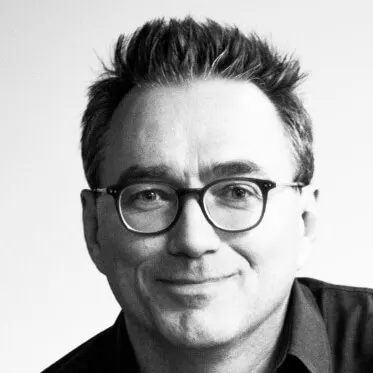
Brian
Ames
Senior Manager of Production AI and Data Products,
General Motors
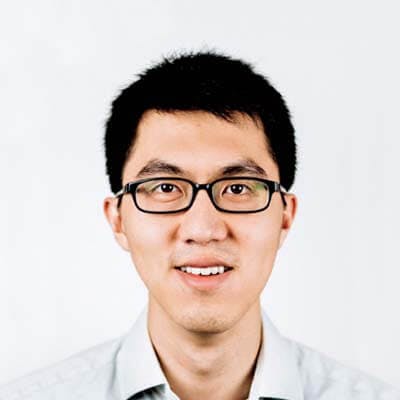
Reynold
Xin
Co-founder and Chief Architect,
Databricks
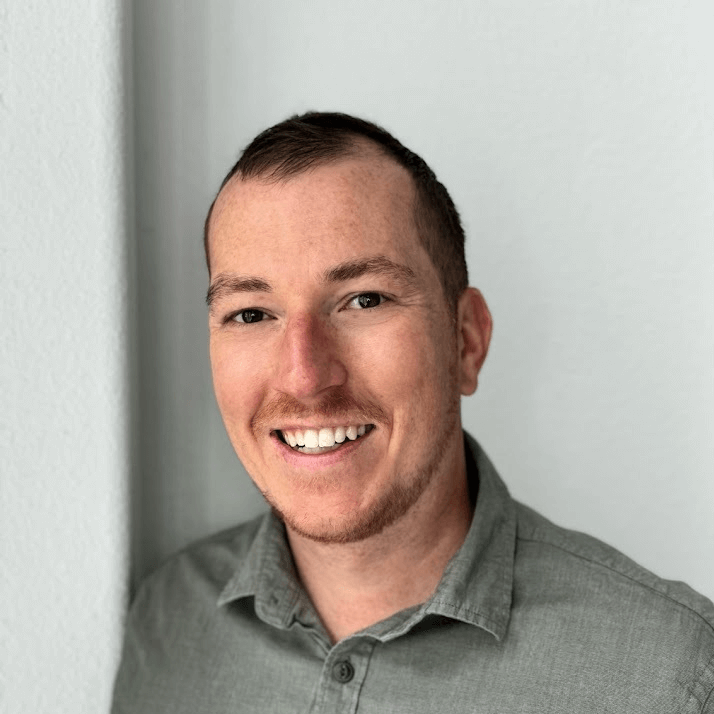
Alexander
Booth
Assistant Director of R&D,
Texas Rangers Baseball Club
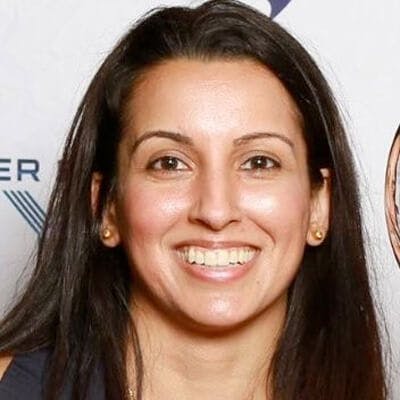
Zaheera
Valani
Senior Director of Engineering,
Databricks
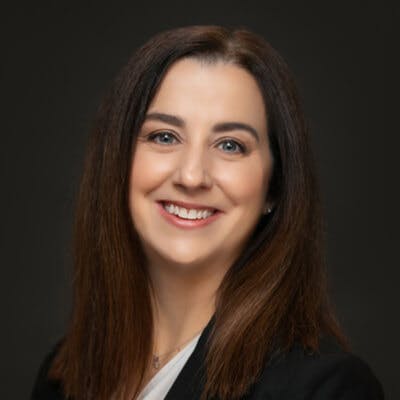
Robin
Sutara
Field CTO,
Databricks
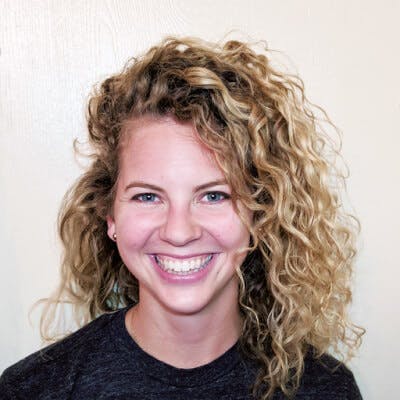
Kasey
Uhlenhuth
Staff Product Manager,
Databricks
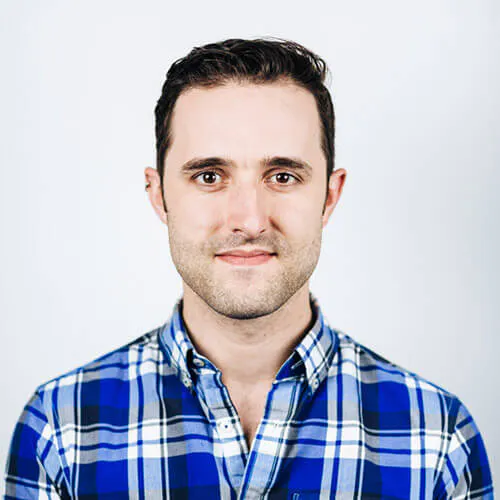
Michael
Armbrust
Distinguished Engineer,
Databricks
SESSIONS
With over 500 sessions covering everything from data warehousing, governance and the latest in generative AI, here are some of the highlights.
AI and the Lakehouse: Shell’s Journey Towards Effective Data Governance
Honeywell Intelligrated’s IoT Streaming Lakehouse
Software 2.0: Shipping LLMs with New Knowledge
WHY ATTEND
Join thousands of data leaders, engineers, scientists and architects to explore the convergence of data and AI.
LEVEL UP YOUR SKILLS
TRAINING AND CERTIFICATION
Whether you’re just getting started or a seasoned pro, we have a course for you.
LEARN
DISCOVER THE LATEST TECHNOLOGIES
Explore the latest advances in Apache Spark™, Delta Lake, MLflow, PyTorch, dbt, Presto/Trino and much more. You’ll also get a first look at new products and features in the Databricks Data Intelligence Platform.
NETWORK
MAKE MEANINGFUL CONNECTIONS
Connect with thousands of data and AI community peers and grow your professional network in social meetups, on the Expo floor or at our event party.
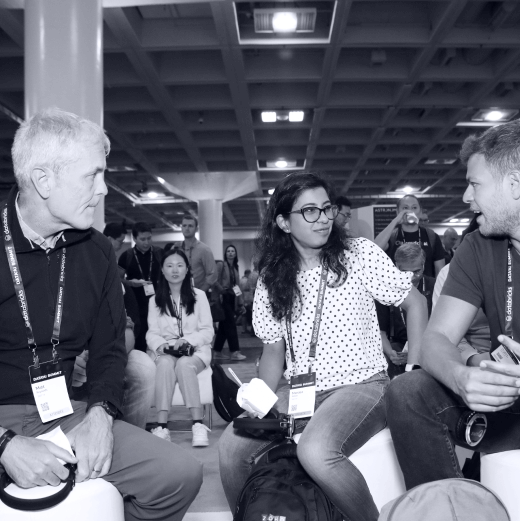
_GENERAL ADMISSION
$1895
SPONSORS
Summit wouldn’t happen without our awesome sponsors. Interested in sponsoring Data + AI Summit? Reach out to our Sponsorship Management team to learn about available opportunities.