Featured speakers
Data + AI Summit speakers include leading experts, researchers and open source contributors — from Databricks and across the data and AI community
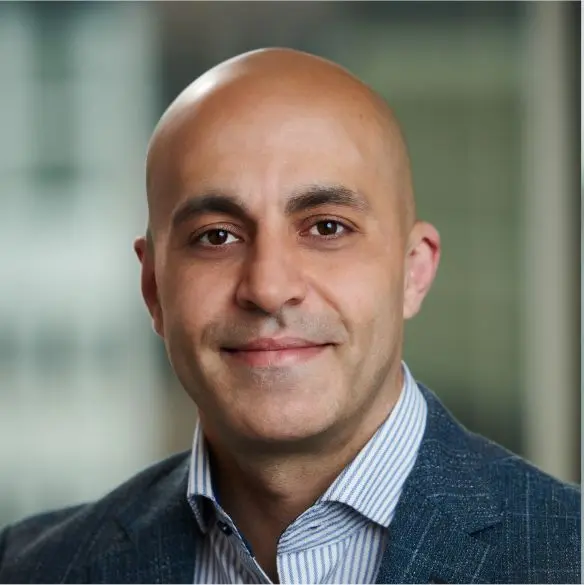
Ali
Ghodsi
Co-founder and CEO,
Databricks

Jamie
Dimon
Chairman and CEO,
JPMorgan Chase
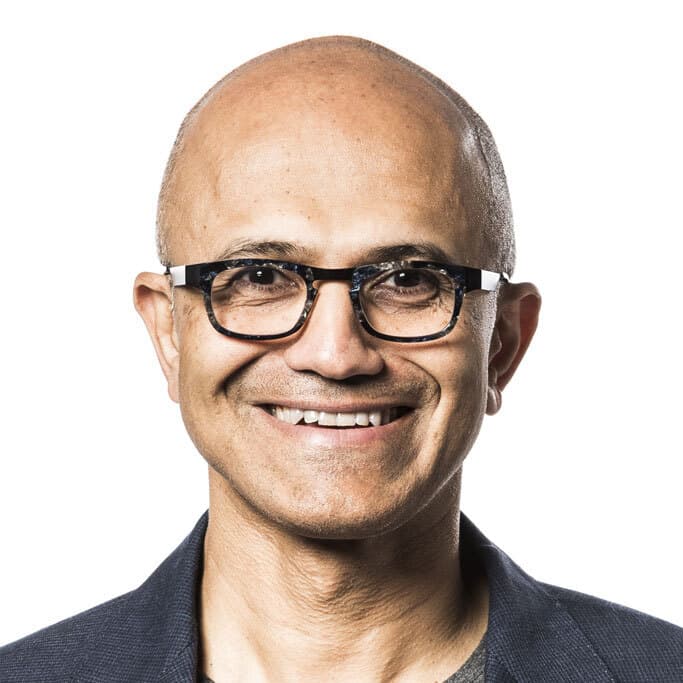
Satya
Nadella
Chairman and CEO,
Microsoft
*pre-recorded
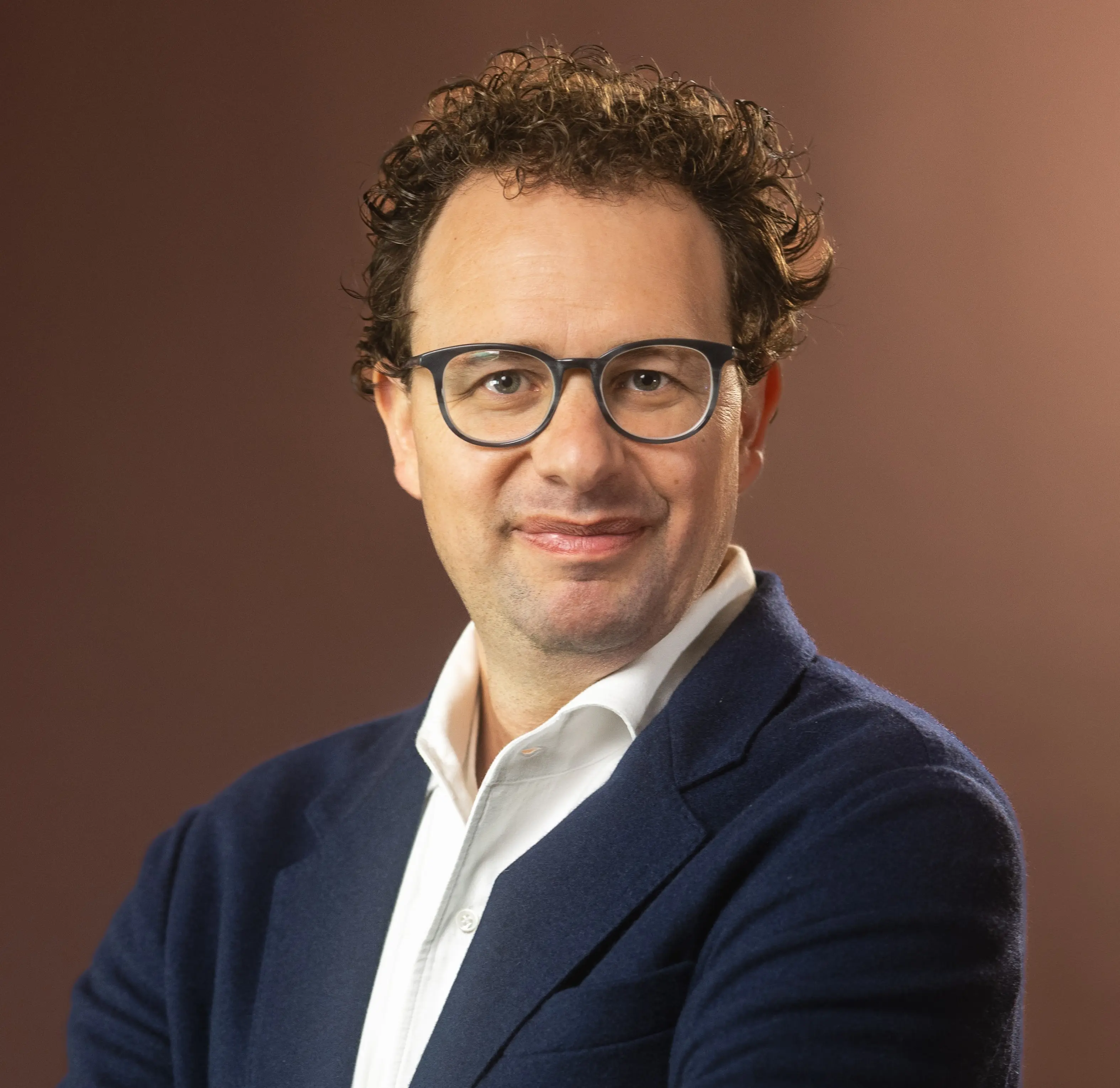
Dario
Amodei
Co-founder and CEO,
Anthropic
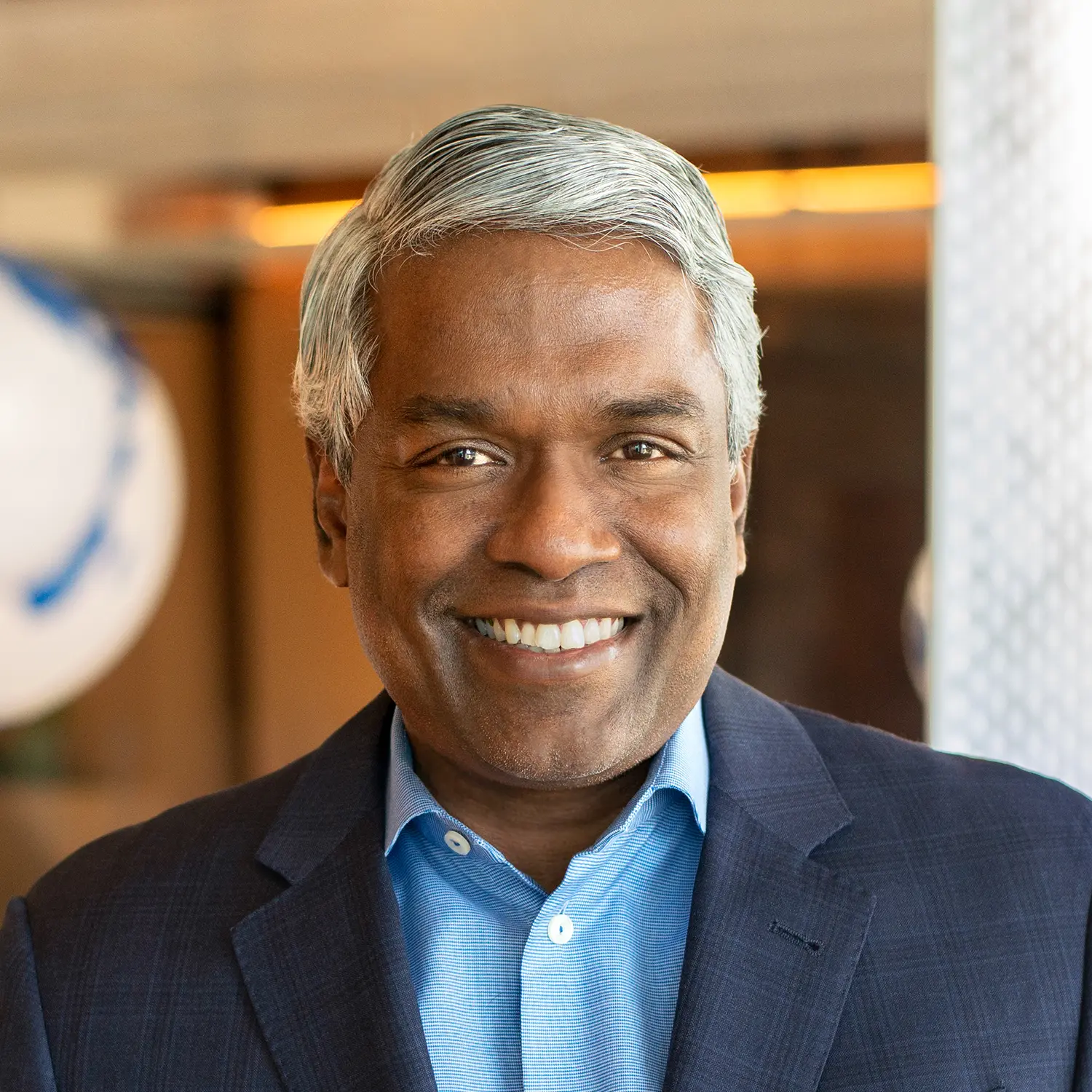
Thomas
Kurian
CEO,
Google Cloud
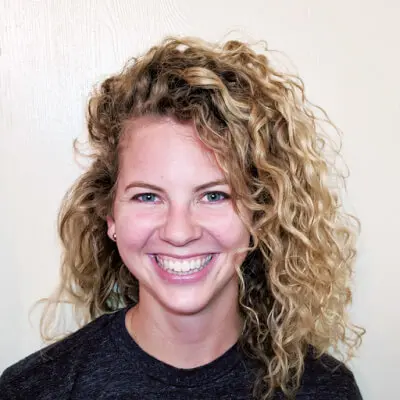
Kasey
Uhlenhuth
Director of Product Management,
Databricks
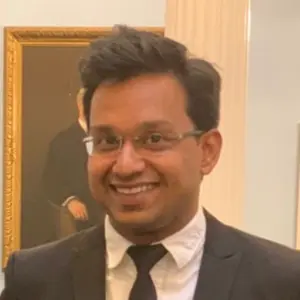
Aditya
Gautam
Machine Learning Lead,
Meta
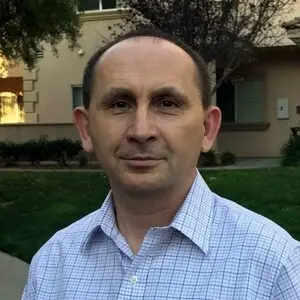
Sergey
Zavgorodni
Lead Data Engineer,
DoorDash
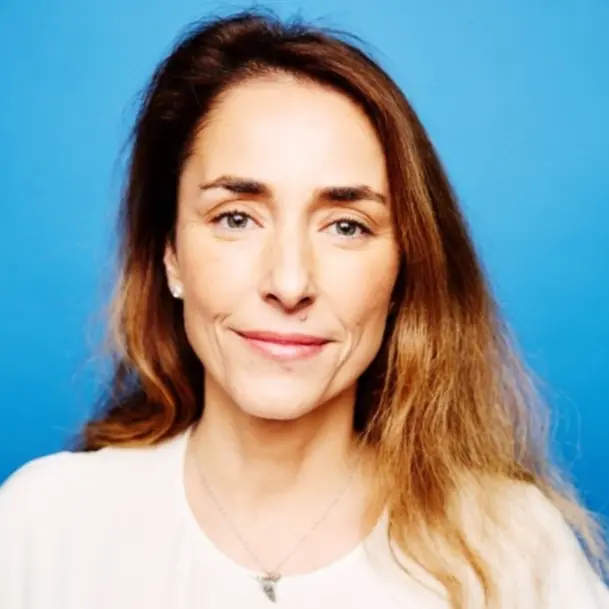
Zeynep
Ozdemir
Chief Marketing Officer,
Atlassian Pty Ltd.
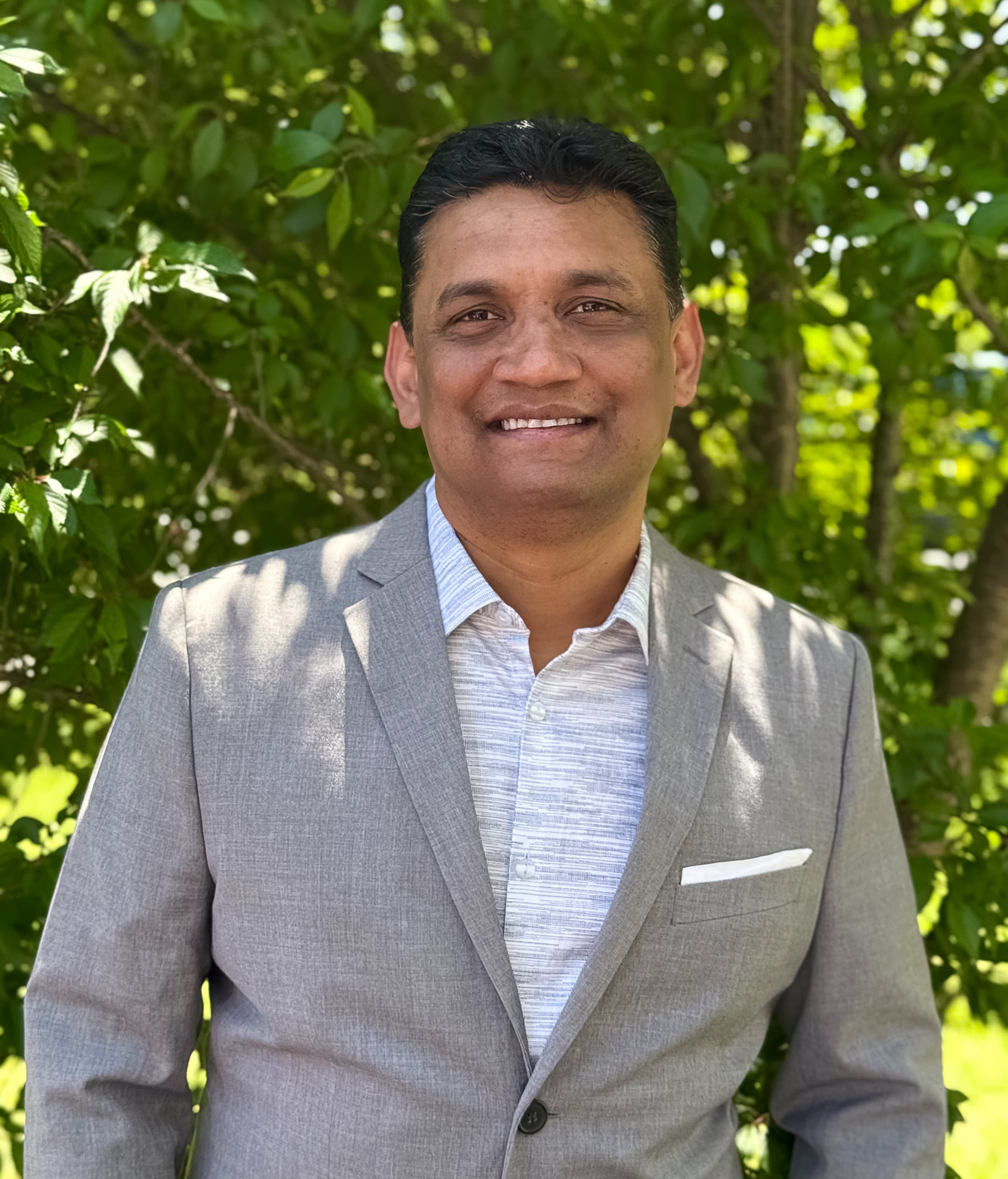
Anil
Chamarthy
Director, Software Engineering,
Capital One
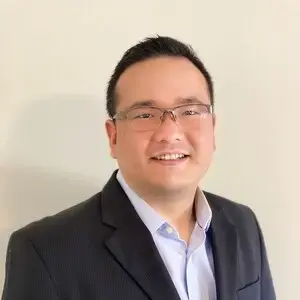
Joshua
Sayah Lee
Lead Global D&AI Solution Architect,
PepsiCo Inc.
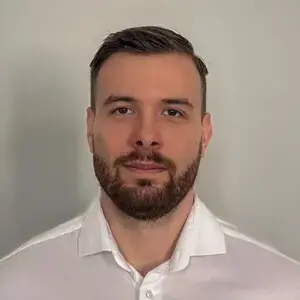
Tomáš
Drietomský
Senior Data Scientist,
Mastercard
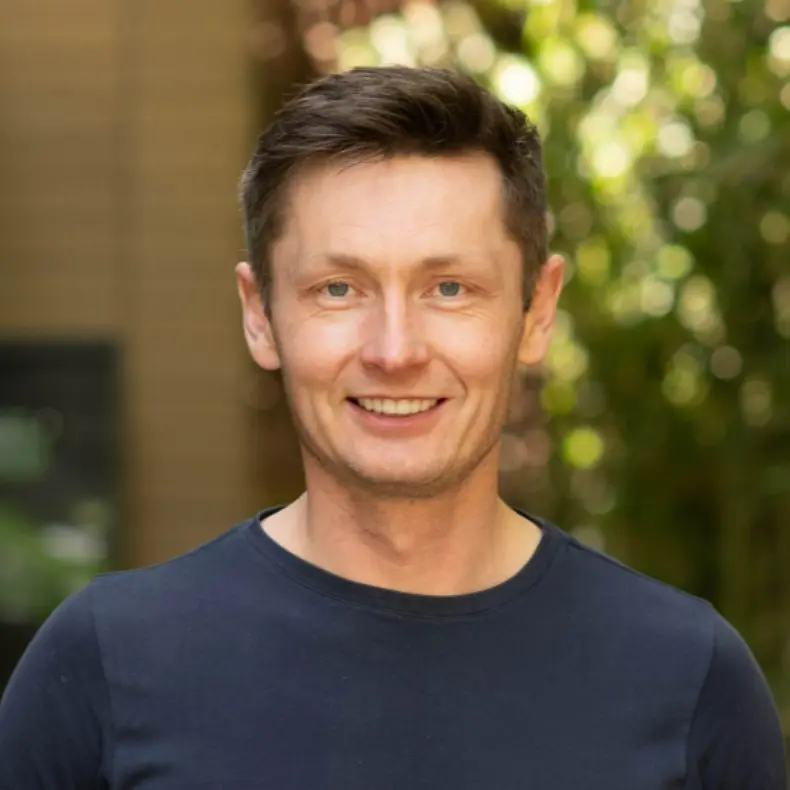
Nikita
Shamgunov
Co-founder and CEO,
Neon
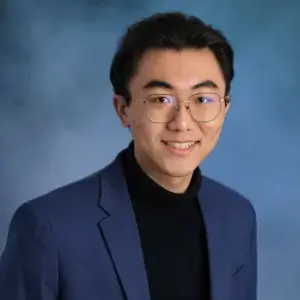
Sihang
Chen
Sr. Machine Learning/AI Engineer,
Rivian
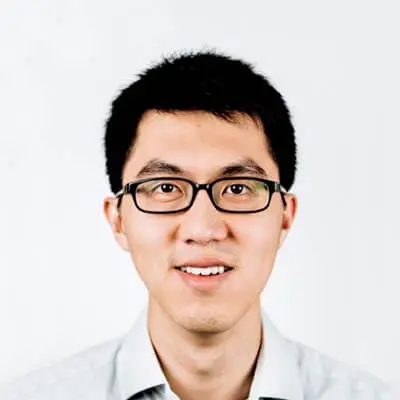
Reynold
Xin
Co-founder and Chief Architect,
Databricks
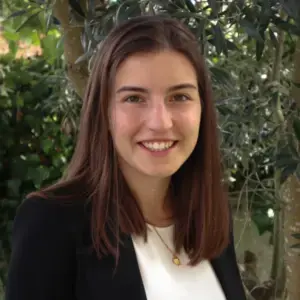
Joana
Ferreira
ML Engineer,
Adidas AG
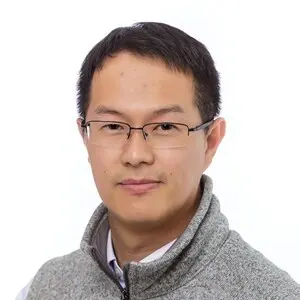
Indra
Rustandi
Staff ML Engineer,
Coinbase
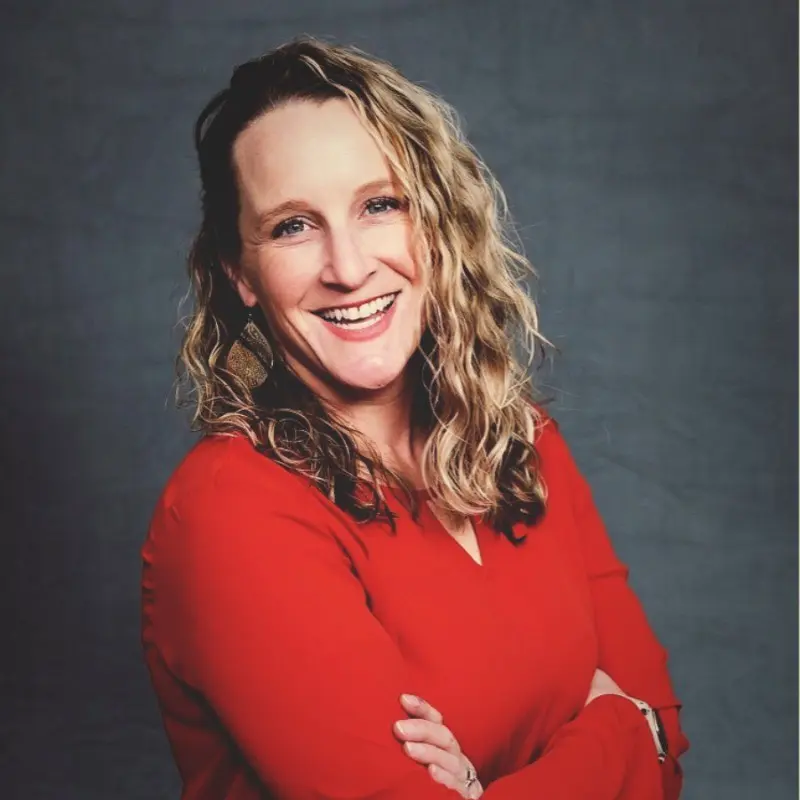
Calen
Holbrooks
Vice President, Marketing,
Airtable
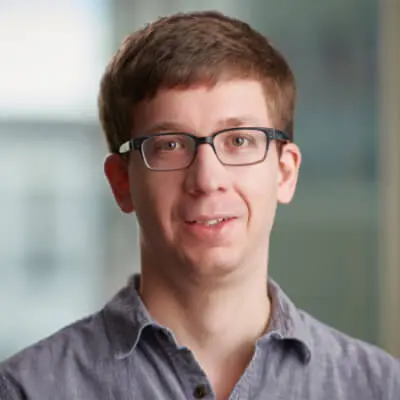
Matei
Zaharia
Original Creator of Apache Spark™ and MLflow; CTO,
Databricks
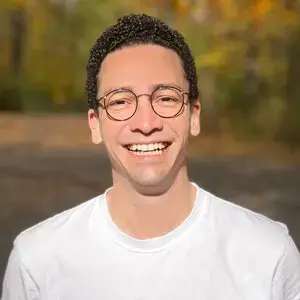
Mohammed
Farag
Senior Manager, Machine Learning & AI,
Rivian Automotive, LLC
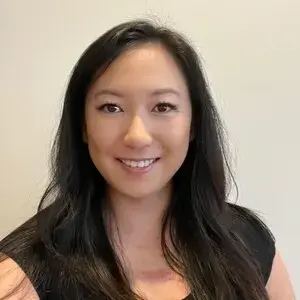
Jiong
Liu
VP of Product Marketing,
Wiz
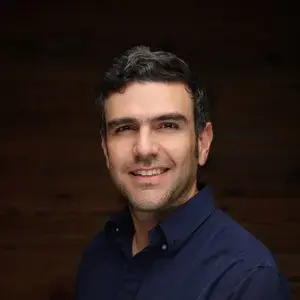
Roberto
Robles Nacif
Director, Product Development,
Walmart Data Ventures
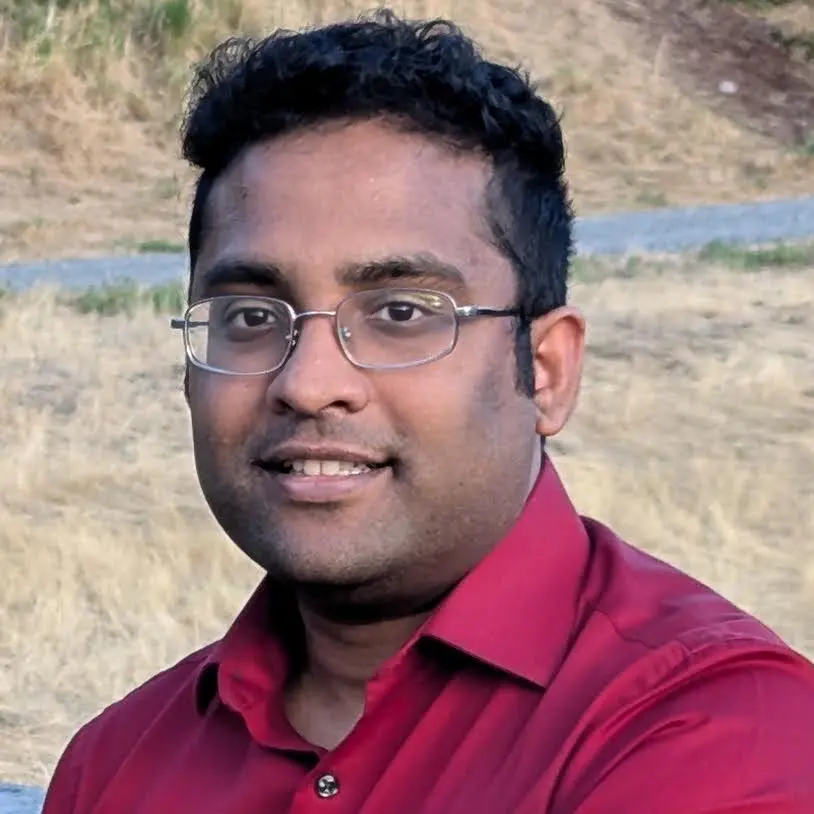
Arvindram
Krishnamoorthy
Principal Architect, ML Solutions,
T-Mobile
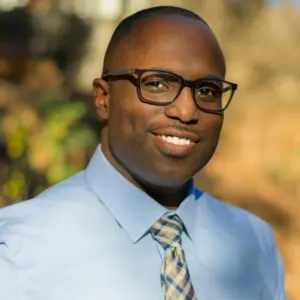
Andrew
Hinton
Principal Data Scientist,
CVS Health
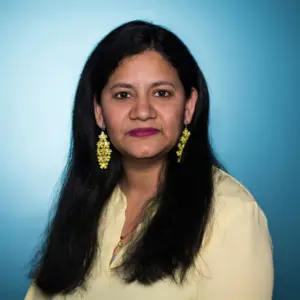
Anuradha
Maradapu
Manager, Data Governance & Engineering,
American Airlines
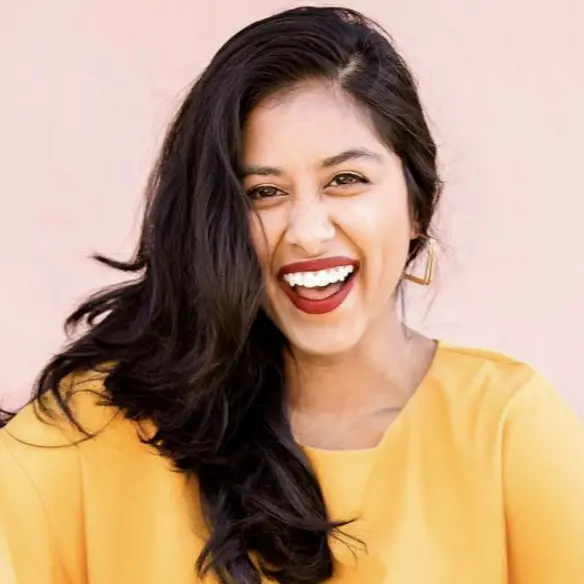
Aparna
Dhinakaran
Co-Founder and Chief Product Officer,
Arize
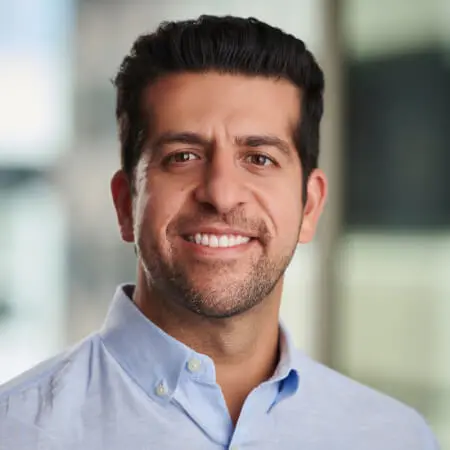
Arsalan
Tavakoli-Shiraji
Co-founder and SVP of Field Engineering,
Databricks
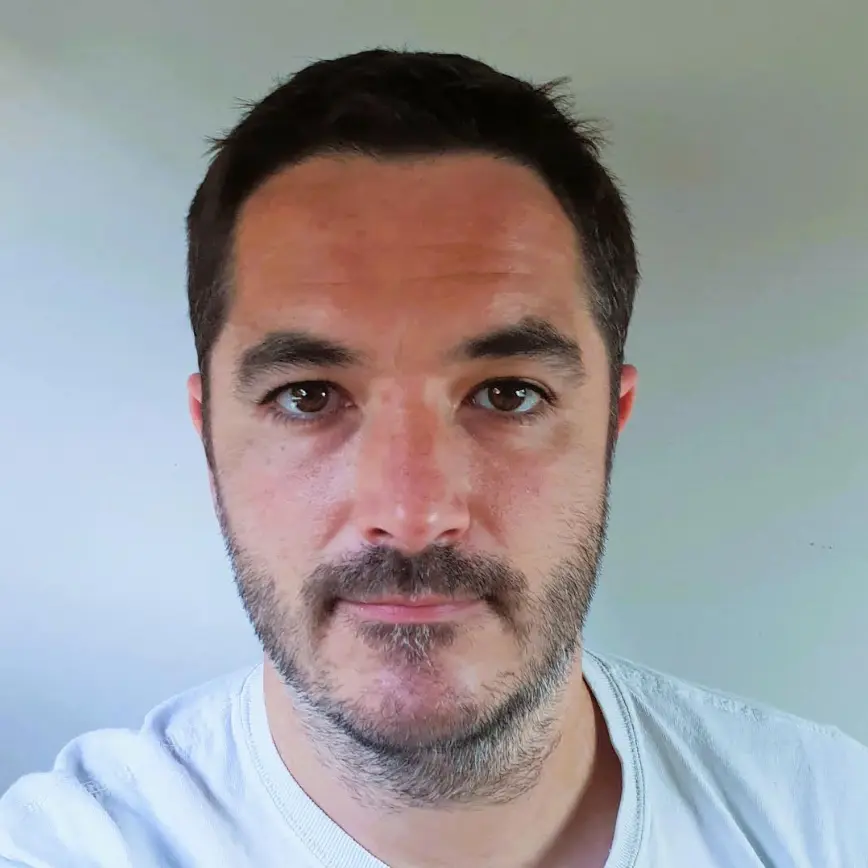
Felix
Baker
Head of Data Services,
SEGA Europe Limited
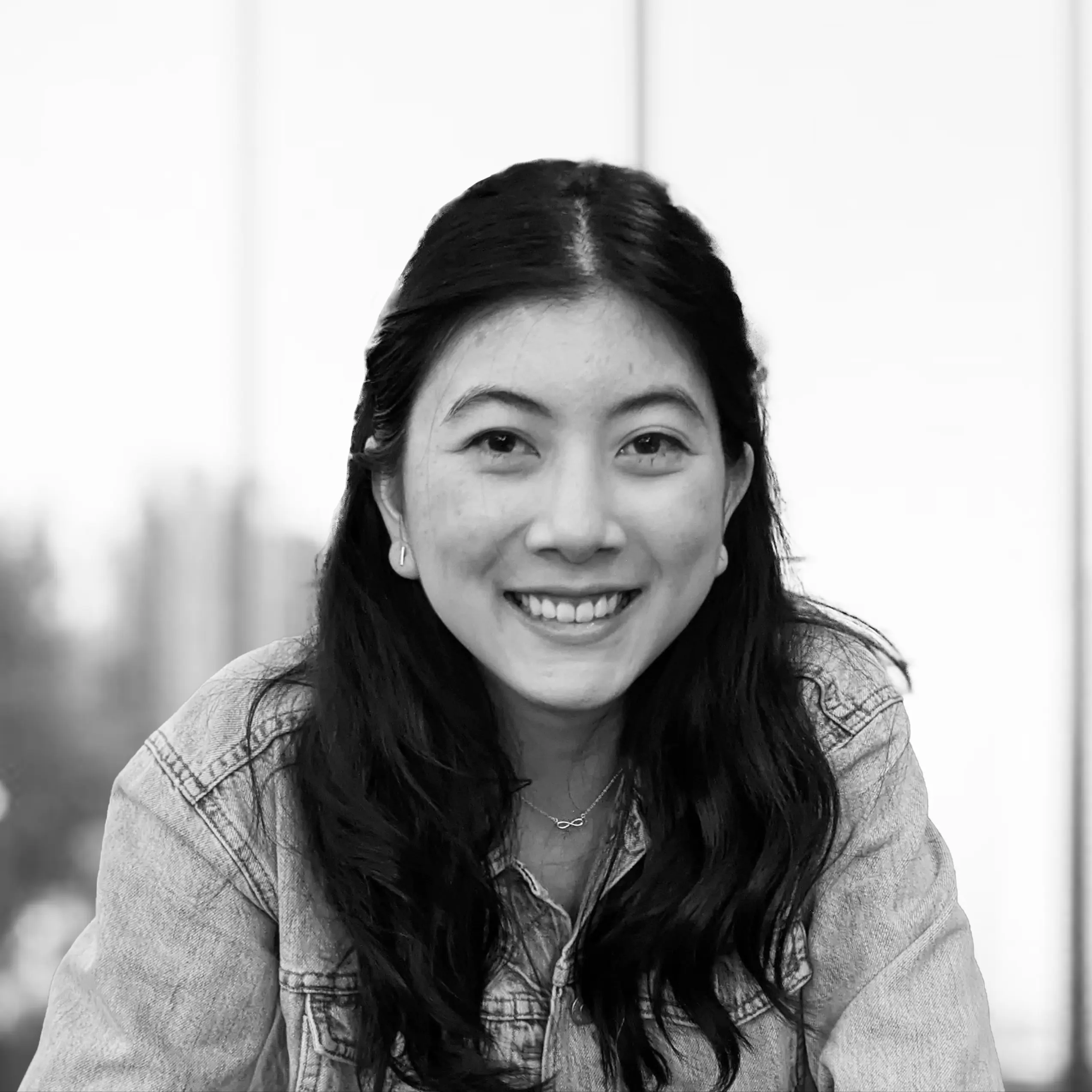
Michelle
Leon
Staff Product Manager,
Databricks
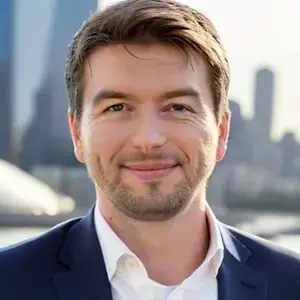
Richard
Masters
Vice President, Data & AI,
Virgin Atlantic
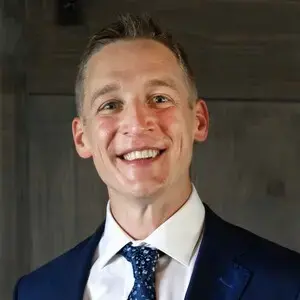
Michael
Flynn
Director, Core Data,
Rivian and Volkswagen Group Technologies
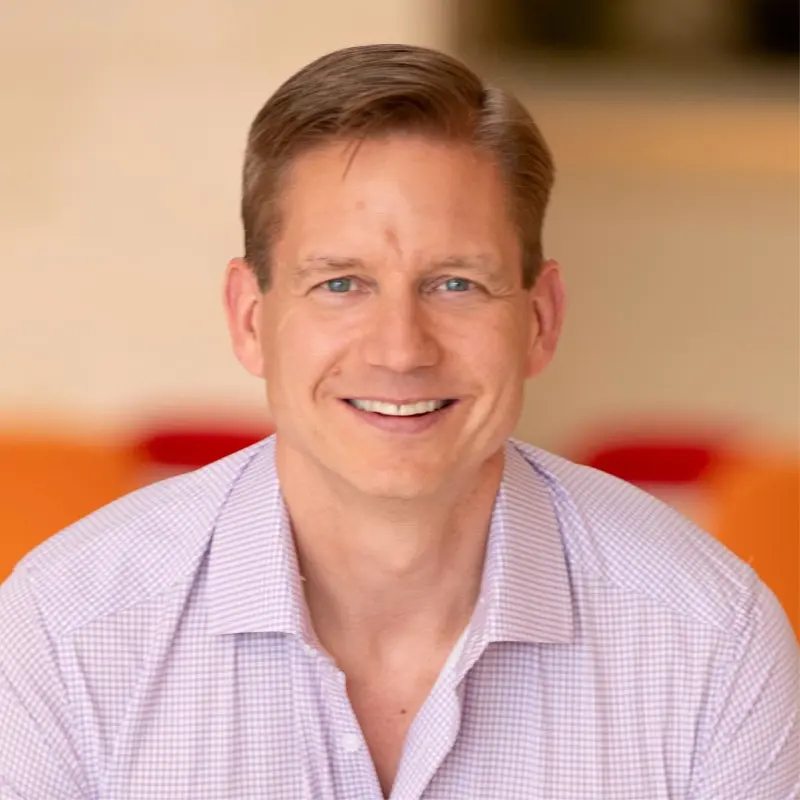
Greg
Ulrich
Chief Data and AI Officer,
Mastercard
Sessions
700+ sessions — from data intelligence to data warehousing, governance to AI. Here are some key highlights.
Self-Service Assortment and Space Analytics at Walmart Scale
Optimizing EV Charging Experience: Machine Learning for Accurate Charge Time Estimation
Evolving Data Insights With Privacy at Mastercard
Powering Secure and Scalable Data Governance at PepsiCo With Unity Catalog Open APIs
Sponsors
Data + AI Summit couldn’t happen without our awesome sponsors. Interested in sponsoring? Reach out to our Sponsorship Management team to learn about available opportunities.