Improve AI Training With the First Synthetic Personas Dataset Aligned to Real-World Distributions
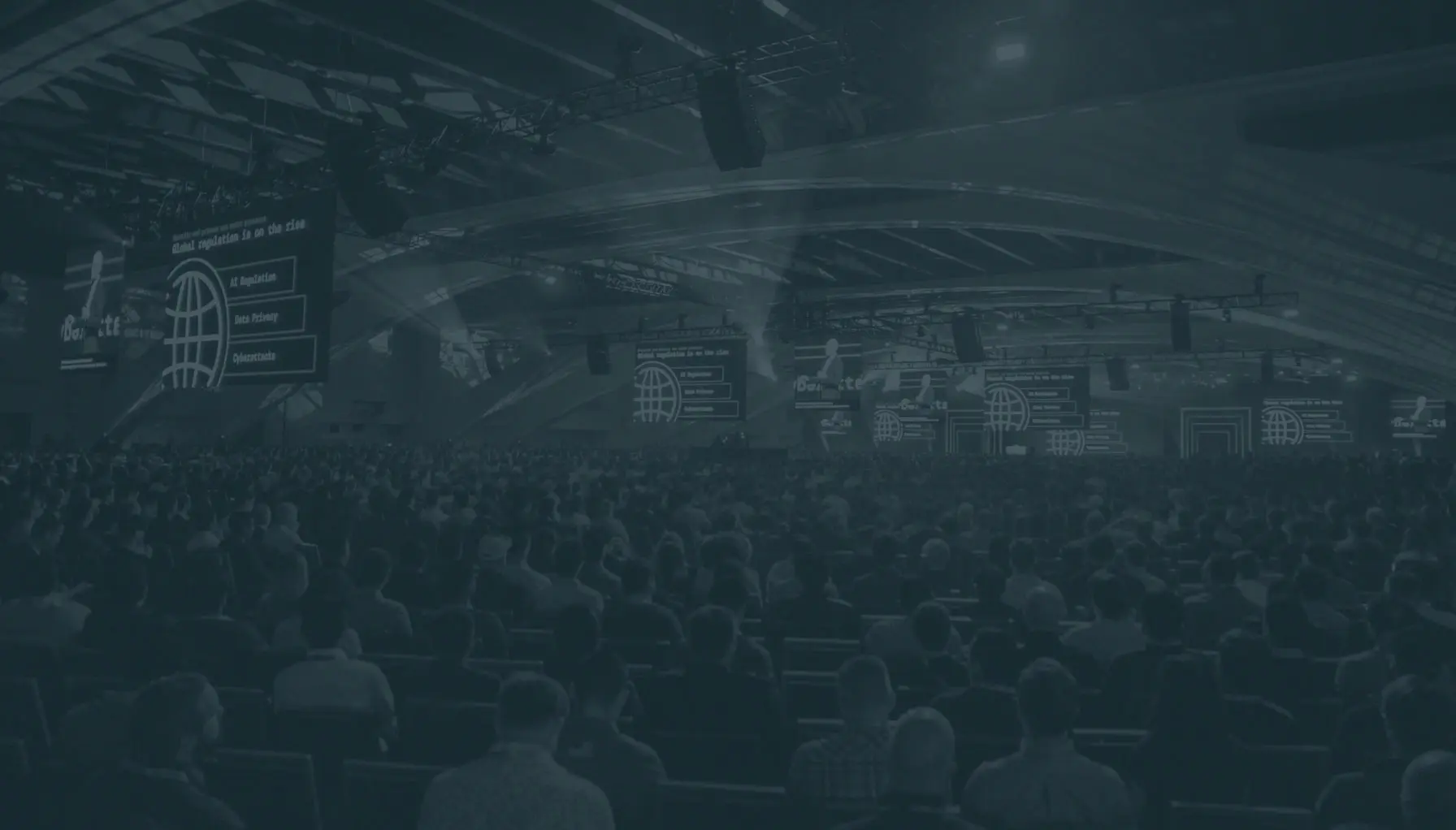
Overview
Tuesday
June 10
8:00 am
Experience | In Person |
---|---|
Type | Breakout |
Track | Artificial Intelligence |
Industry | Enterprise Technology, Health and Life Sciences, Financial Services |
Technologies | Apache Spark, AI/BI, Llama |
Skill Level | Intermediate |
Duration | 40 min |
A big challenge in LLM development and synthetic data generation is ensuring data quality and diversity. While data incorporating varied perspectives and reasoning traces consistently improves model performance, procuring such data remains impossible for most enterprises. Human-annotated data struggles to scale, while purely LLM-based generation often suffers from distribution clipping and low entropy.
In a novel compound AI approach, we combine LLMs with probabilistic graphical models and other tools to generate synthetic personas grounded in real demographic statistics. The approach allows us to address major limitations in bias, licensing, and persona skew of existing methods. We release the first open-source dataset aligned with real-world distributions and show how enterprises can leverage it with Gretel Data Designer (now part of NVIDIA) to bring diversity and quality to model training on the Databricks platform, all while addressing model collapse and data provenance concerns head-on.
Session Speakers
Dane Corneil
/Senior Staff Applied Scientist
NVIDIA
Yev Meyer
/Principal Research Scientist
NVIDIA