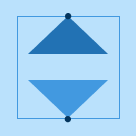
Artificial Intelligence (AI) is going to be embedded in every product and service a business produces and customers interact with. With Generative AI, we're now entering an era of higher expectations of data & AI initiatives contributing to the competitive advantage of any company. Data Governance is absolutely critical to get right if a company is to succeed in creating and sustaining a competitive advantage. The significance of data governance today cannot be overlooked in today's dynamic context - it is a necessary imperative to deliver AI initiatives. Why? Because good AI comes from good data. Without proper governance, you cannot ensure good data.
Yet, data governance has one major problem. To best describe it, I'll take the queue from the cheerfully scary Hollywood character, Inigo Montoya (of Princess Bride fame), "Data Governance…. You keep using the word. I do not think it means what you think it means!". It's a funny yet shockingly accurate representation of the reality that the term has become so amorphous that organizations struggle to define what it is, how it can create value accretive outcomes for the business and how it is distinct from compliance initiatives.
To illustrate how data governance impacts every facet of an organization and why it matters to key stakeholders (in particular those with AI applications), let's follow the lifecycle of a product, processes, and the people whose work is impacted by a strong data governance strategy.
Delivering better customer experiences with better data
Imagine your installed base of connected products continuously streaming important health and user interaction information to your company's data platform. Your customer support team wants to build an LLM model and chatbot that enables service agents and field engineers to prioritize the right issues and recommend the right solutions. The quality of decisions that customer-facing teams make based on this information is highly sensitive to the quality of this data, particularly completeness and timeliness.
For example, service teams looking to understand which parts of the fleet are running on the latest configuration, identify segments that are seeing degradation in performance, and simulate the impact of possible recovery strategies. Additionally, field engineers use this information to understand reliability trends and the best possible solutions that can deliver the best economic value to the customers. The cost of poor data quality in these scenarios can lead to suboptimal decisions that can cost companies millions of dollars per event, in addition to an erosion of customer trust.
Governance has to be tightly integrated with data management. Enforcing strict data integrity checks at every step is paramount to making better decisions as a customer-centric organization, keeping products in the best operating condition, and delivering the best customer experience.
By ensuring visibility into data integrity checks at every step of the data value chain, companies can achieve better synchronization, faster root cause analysis, and a more accurate assessment of downstream data products such as predictions, reports, dashboards, and GenAI apps that are consumed by end-users.
Orchestrating agile supply chains through seamless data collaboration
Imagine your supply chain team wants to use data science techniques to predict the market demand for your products more accurately - enabling the business to optimize inventory levels, and design more precise replenishment and production plans. They envision the ability to automate more of their logistics and warehouse operations to reduce errors, increase on-time delivery performance, and make smarter capital allocation decisions. More accurate, complete, and timely forecasts depend on collaboration between different functions from the supply chain, procurement, finance and operations, business units, and even external agencies such as suppliers, distributors, and logistics partners.
Good data governance practices fosters collaboration, not hinder it. It ensures that everyone has the right data to make better decisions. Strong, reliable data-sharing amongst both internal and external stakeholders delivers more accurate and meaningful analytics. Without it, each function creates their own version of reality vs. devising a more complete picture of the operation. In order to build a robust and sustainable supply chain, companies need an even stronger focus on data governance that interlocks seamlessly across their entire business and ecosystem.
Manufacturing companies require a governed approach to sharing data, both within their organization (across different departments and lines of business) and externally (with suppliers, trading partners, and dealers/distributors). This is necessary to gain a more comprehensive and real-time understanding of the factors that can impact their operational and supply chain performance.
Smarter manufacturing with governed AI
Quality requirements in almost every corner of the industry are increasing with tighter emission regulations and customer expectations. Imagine a computer vision-based defect detection model that helps quality control professionals identify and scrap potentially defective products earlier in the production process so that valuable manufacturing resources are not wasted and more importantly, defective products do not end up in the hands of customers.
Industrial AI systems for decision-making will be trained mostly on unstructured data from sensors, images, videos, text, documents, and complex systems. With mission-critical use cases that impact safety, quality and productivity, the cost of poor predictions can cost millions of dollars. With the stakes in industrial AI so high, bad data is not a recipe for success. The promise of AI cannot be realized with shortcuts in governance. The industry needs a comprehensive approach towards governance that starts with data management and extends to the end-to-end development of AI.
With the criticality of problems being solved by AI in Manufacturing, the industry needs a more comprehensive approach to govern the entire AI workflow across all data types, features, and models to improve explainability, traceability, and reproducibility over the lifecycle of these data and AI assets.
Design better products with comprehensive data discovery and lineage
The product cycles in the industry are getting exponentially shorter. The infusion of software and AI in core products requires a different approach that can unify datasets across different parts of the product life cycle ranging from design, manufacturing, service, and optimization. However, there is still a large technical skill barrier for domain experts to interact with data platforms that hold this valuable information.
The most immediate application of Generative AI is to continuously learn the structure of your data to match your company's unique organizational structure, specific acronyms, and product terminology, providing users, regardless of skill level, a natural language interface to discover the right datasets and deliver insights specific to their business. One area that will benefit from this is engineering simulations and workflows, which can now take advantage of AI models to leverage data from real-world environments, streamline repetitive tasks in design processes, and foster stronger data collaboration amongst cross-discipline teams.
A comprehensive approach to data lineage that spans the entire lifecycle from data origination to usage, brings the trust, traceability, and ability to audit necessary to unlock this next wave of engineering productivity. Ultimately, this enables organizations to iterate on better designs faster and cheaper than previously possible.
Looking ahead: Unlock data & AI democratization with more effective governance
Ultimately, a company's data and AI strategy is about making better decisions. Effective governance of Data and AI is a pathway to making better decisions, and not a hindrance, across every step in the value chain and every corner of the organization. We believe that companies that take a more comprehensive approach towards governance will be the best at creating a strong competitive advantage with their data. In this golden age of AI, there are five questions any executive should ask to inform their next steps on governance.
- Data Quality: The data in our industry keeps getting more unstructured and diverse (applications, IoT devices, telemetry, images/video, etc.). How does the company scale its data curation processes and deliver high-quality data products to a broader range of users amidst this increasing complexity?
- Governance of AI: Most AI work takes place in the realm of unstructured data. Does the company's strategy address the governance of artifacts in the end-to-end development of AI (e.g. features, models, unstructured data)?
- Collaboration: There is a constant need to democratize information to multiple departments: marketing, aftersales, operations, manufacturing, R&D, and even external business and supply chain partners. How does the company's approach to governance enable this level of collaboration with internal and external stakeholders?
- Security: The landscape of contractual, legal, regulatory, and industry practices around AI is ever-increasing. What measures do we have in place to more confidently demonstrate that the use of data & AI inside our company is aligned with market and industry expectations?
- Reproducibility: AI is powering time-sensitive decisions that drive tangible real-world outcomes in safety, reliability, efficiency and productivity. As the pace of innovation and complexity of models increases, how is the company gaining a more comprehensive view of end-to-end data lineage to improve the explainability & reproducibility of their AI systems over time?
To learn more about governance, generative AI and the Databricks DI platform, please leverage the following resources: