Migrate to Databricks
Modernize your data platform by moving to the Databricks Lakehouse
Reduce costs, innovate faster and simplify your data platform by migrating to the Databricks Lakehouse from your enterprise data warehouse or legacy data lake. Now you can run all your data, analytics and AI workloads on a modern unified platform, built on open standards and secured with a common governance approach.
Why migrate to Databricks?
Simplify your data platform
Get a single modern platform for all your data, analytics and AI use cases. Unify governance and the user experience across clouds and data teams.
Scale cost-effectively
Stop managing servers, and scale on demand with serverless. Run data warehousing at scale with up to 12x better price/performance.
Accelerate innovation
Build AI, ML and real-time analytics capabilities faster with collaborative, self-service tools and open source technologies such as MLflow and Apache Spark™.
Migrate with confidence
We’ve helped hundreds of customers migrate from legacy data platforms. Using a phased end-to-end migration process provides a predictable model to understand costs both during and post-migration. And a lakehouse-first approach for migrating all workloads ensures support for existing as well as new use cases. The result is lower risk, faster value realization and increased ROI.
The 5 phases of data migration
Phase 1:
Discovery
Use profilers to automate discovery. Get insights on legacy platform workloads and estimate Databricks platform consumption costs.
Phase 2:
Assessment
Use analyzers for a detailed assessment of code complexity and to estimate migration project costs.
Phase 3:
Strategy
Finalize technology mapping and build optimal pathways for each source platform migration, with expert guidance from Databricks.
Phase 4:
Production Pilot
Run a pilot with your use cases and use code converters where applicable to transpile legacy code to Databricks-compatible code. Develop a migration implementation plan and road map.
Phase 5:
Execution
Rinse and repeat for all workloads. Get help with migration execution and support from certified partners or Databricks Professional Services.
Customers who have successfully migrated to Databricks
Brickbuilder Solutions
Leverage Brickbuilder Solutions from leading consulting partners — built for migrating to the Databricks Lakehouse
Cloud Data Migration by Accenture
Less guesswork, more value
Legacy System Migration by Avanade
Move your data to unlock its full value
Migrate to Cloud and Databricks by Capgemini
Streamline data migration to the Databricks Lakehouse Platform
Migrate to Databricks by Celebal Technologies
Quicker migration from on-premises at lower cost
Migration Factory by Deloitte
A holistic approach to cloud data migration
LeapLogic Migration Solution by Impetus
Auto-transform ETL, data warehouse, analytics and Hadoop workloads to Databricks
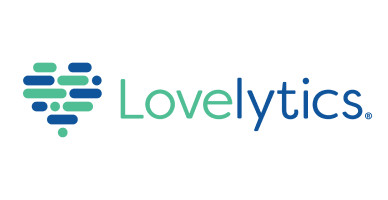
Snowflake-to-Databricks Migration by Lovelytics
Ensure a rapid and sound migration process
Migration Factory by Perficient
Modernize your data platform with a focus on architectural and process efficiencies
Data Intelligence Suite by Wipro
Migrate with confidence to Databricks
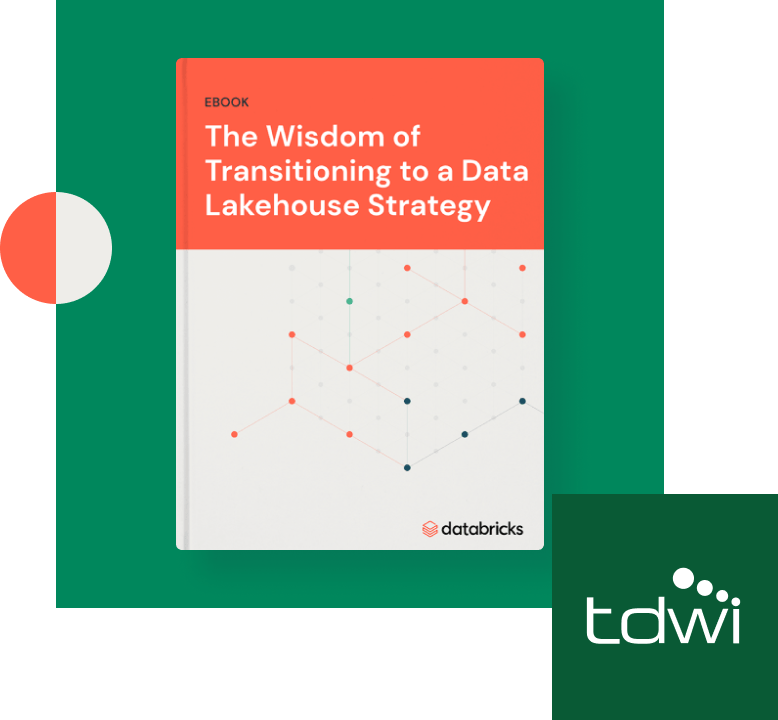
The Wisdom of Transitioning to a Data Lakehouse Strategy
How a data lakehouse approach can help you overcome the common limitations of data warehouses and data lakes
Resources
eBooks
Events
Blogs
Get started
Migration doesn’t have to be a headache. Contact us today to talk about what it could look like for you.