Driving toward peak operational efficiency
Michelin uses Databricks to streamline business operations
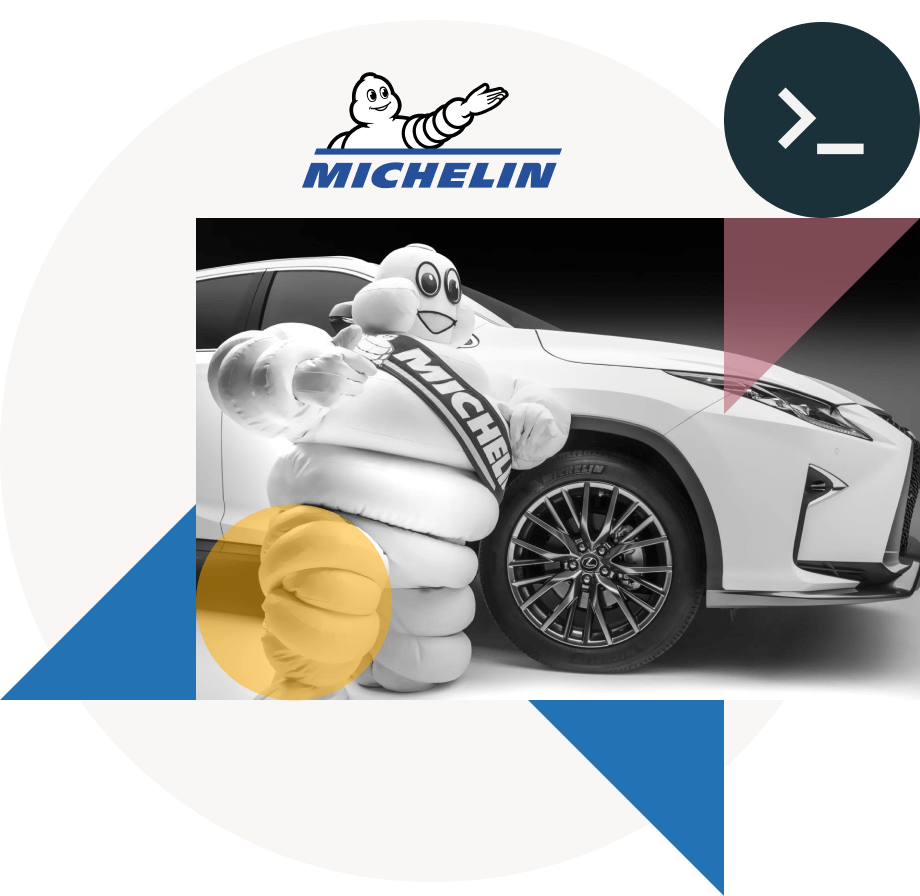
Michelin doesn’t just make tires. They are paving the path toward the future, constantly rolling out new innovations that not only move people forward but also steer the automotive industry in new directions. As part of their shift toward becoming a data-driven organization, the company looked to democratize data from diverse sources, from back-office systems to manufacturing sites, unlocking its value so their community of “citizen users” and distributed IT teams could develop their own use cases — such as using AI to predict stock outages and to reduce carbon emissions in the supply chain. Their centralized legacy data platform, however, made it difficult for their increasingly decentralized users to access the tools and technologies they required. With the Databricks Data Intelligence Platform, Michelin is now able to offer every employee access to complete and reliable data from which they can develop use cases that will create value for the business and their customers.
Data democratization hindered by lack of openness and flexibility
A key element of Michelin Group’s transformation initiative is to empower everyone within the organization to have unfettered access to actionable data. This will generate value across every facet of the business, including optimizing supply chains, predicting stock outages, financial forecasting and market intelligence.
To enable this, Michelin built a data transformation strategy around three pillars. The first was the creation of a platform to provide each employee and feature teams with a secure workspace and access to largely decentralized data with which they could build their own use cases. The second pillar concerned governance: helping the business understand how to make data accessible in a secure manner and coordinating the different efforts. The third encouraged every digital solution team to think about their data as a product to be consumed and ensure it was well described, accessible and trustworthy.
“We believe in the democratization of data, a self-serve approach where users can come to a platform, build a new data product and then run it,” said Joris Nurit, Head of Data Transformation at Michelin. “We wanted to ensure data was accessible to Michelin’s employees and to make the right tools available so users could solve their use cases by themselves.”
However, Michelin’s former on-premises data platform lacked the necessary openness and flexibility. With data siloed by department and the centralized nature of the platform, providing services at scale was challenging, and employees were often unable to access the tools and technologies they needed. A scalable, flexible and — importantly — open platform was needed. And for this, Michelin turned to Databricks.
Lakehouse unlocks the value of data across the entire organization
Allowing employees to access Databricks directly through their notebooks to perform their own transformations and analytics has allowed Michelin to realize their vision of building a “citizen data platform.” The introduction of a central lakehouse enabled Michelin to break down the data silos that previously existed between different departments while ensuring significant autonomy for the teams building centralized data products.
Nurit commented, “By mixing their own local data with the central data, each team can gain a 360-degree understanding of their own market. This allows them to better anticipate the needs of their stakeholders and design their use cases accordingly.”
An early use case involved employing AI to predict the shortage of stock. Using machine learning models trained on past supply chain data, the application was designed to predict stock levels in 15 days’ time and recommend actions to prevent outages from occurring in the future. Michelin used Databricks for both the unification of the input data, collaboration on how to apply the data to train machine learning models, and the deployment and orchestration of models into production. And with real-time capabilities, the data itself was continuously streamed and kept up-to-date in Databricks Delta Lake while business analysts used Databricks SQL to run queries and visualize insights.
The number of use cases has grown considerably since then. “Using lakehouse means we’ve been able to scale to hundreds of use cases on the platform, regardless of what they’re focused on. In fact, we don’t know what 80% of them are, and that’s fine,” said Karim Hsini, Enterprise Architect at Michelin. “This way, we can focus on our primary use cases while still having the flexibility to support emerging initiatives that may arise.”
Consuming data held on the Databricks Data Intelligence Platform and deriving insight from the results of its analysis means users can address simple use cases like business reporting or forecasting. It enables more specialized and industrialized use cases, too, addressing the needs of various personas — from citizen data analysts to data scientists.
Autonomy and collaboration lead to a community of innovators
The platform approach is essential. Flexible and scalable, it means every user in the company can develop their own use case with full autonomy. Michelin’s move to the cloud was an important factor in this. Hsini explained, “The modularity of the cloud allows the pre-configuration of an isolated environment for everyone, meaning all users can work without breaking what their colleagues have done.”
This autonomy has led to widespread adoption of their citizen data platform, a growing community of users that has produced greater collaboration within the company. Nurit considers this idea of community a key success factor for the future of Michelin’s innovation.
“Community is important to avoid people reinventing the wheel every time an issue arises,” Nurit concluded. “People share data; they share their code and best practices, working together to solve different use cases. It’s an important step toward Michelin’s ambition of being a data-driven organization. By democratizing data in this way, Databricks has provided a platform which allows their citizen users to develop together.”
-
Learn more about the Michelin Group
-
Follow Michelin's IS and digital teams on LinkedIn