Scaling AI innovation to drive revenue, efficiency and growth
Mondelēz accelerates AI across sales execution, marketing, consumer-centric supply chain and revenue growth management with Databricks
Models managed in batch and real time using MLflow
Models in production using Model Serving
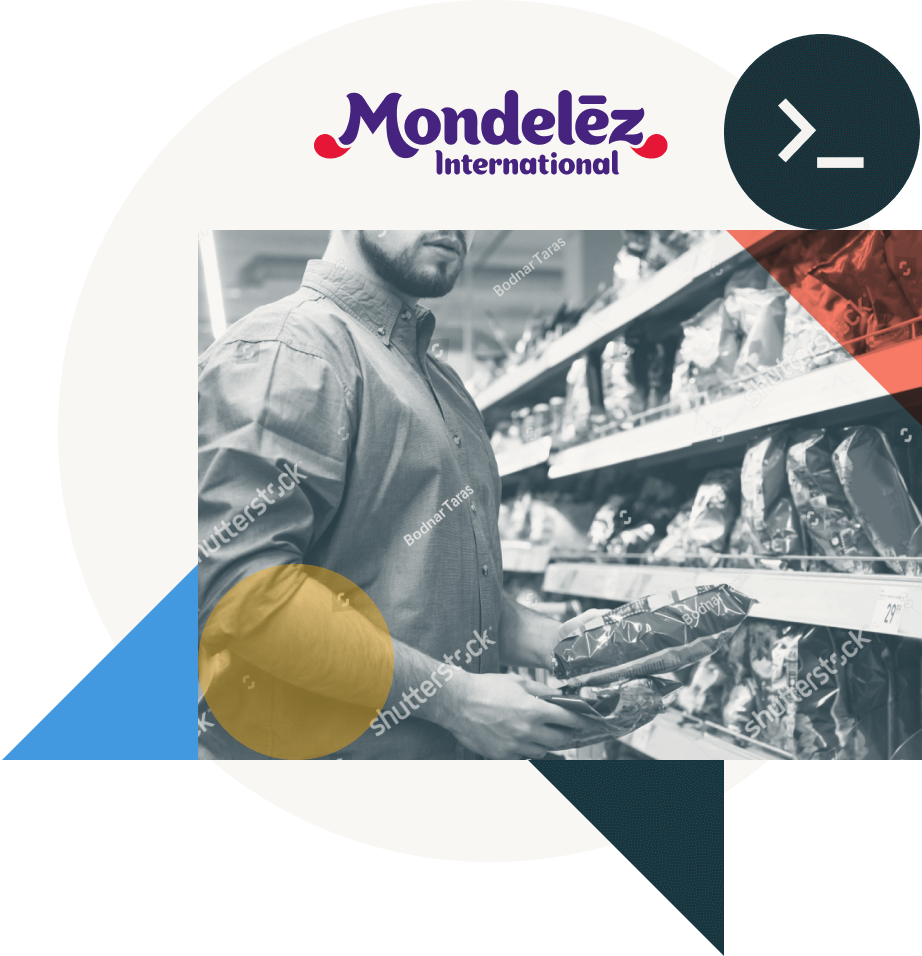
Mondelēz International, maker of globally beloved snacking brands like Oreo, Ritz and Toblerone, operates in more than 160 countries — making the stakes for data-driven decisions incredibly high. With the ambition to become a native AI company by 2030, Mondelēz faced mounting challenges: siloed data, duplicated model development and limited scalability that stifled experimentation and innovation. Enter the Databricks Data Intelligence Platform. By unifying data, AI and governance on a single platform, Mondelēz unlocked new levels of collaboration, accelerated model experimentation across 20,000 models and scaled the production use cases in sales execution, marketing, consumer-centric supply chain and revenue growth management. What once required cobbled-together pipelines and manual oversight is now powered by real-time intelligence — laying the foundation for generative AI, continuous optimization and enterprisewide transformation.
Siloed data and scale limitations leave analytics half-baked
Mondelēz International, one of the world’s largest snacking and confectionary companies, has set a bold ambition to become an AI-driven organization by 2030. With iconic brands like Oreo, Ritz, Toblerone, Cadbury and Sour Patch Kids that all need to operate seamlessly under the Mondelēz umbrella, they are investing in modern data infrastructure and AI-powered intelligence to transform their operations. “We want to leverage AI for product improvements, process efficiencies, and to drive responsible AI practices across the organization,” Sachin Kulshrestha, Global ML Engineering and Ops Lead at Mondelēz, explained. To realize this mission, the company is applying ML and analytics across multiple business domains, including sales execution, marketing, consumer-centric supply chain, revenue growth management and sustainably sourced cocoa and wheat.
Mondelēz uses SKU recommendation models to help sales reps deliver the right products to the right retailers. This has led to a 2–4% increase in store-level topline sales and improved sales rep effectiveness to around 80%. These models evaluate store “potential” by analyzing factors such as geography, demographics and store layout, then cluster stores by similarity to recommend smarter product placement in stores. Route optimization also plays an important factor in improving field sales representative efficiency. “The idea is, when the sales rep goes into the market, what is the route they should choose to maximize coverage? That’s where machine learning gives us an advantage,” Sachin said.
SKU demand forecasting models help the Mondelēz supply chain teams plan from six weeks to two years ahead, supporting both short- and long-term supply network decisions. As a result, Mondelēz reduced finished goods and plant inventory waste by 2–3% and improved forecast accuracy by 3–5%, directly improving operational margins. As Sachin noted, “You don’t want to produce more than you can’t sell. Waste is a revenue loss, both in terms of missed sales and the cost to discarded unsold product.”
Meanwhile, pricing simulations, promotional uplift forecasts and volume transfer modeling help Mondelēz understand how pricing changes may influence consumer behavior and impact overall revenue, volume (lbs/units) and gross profit. This approach has helped Mondelēz identify additional revenue opportunities and improve promotional effectiveness. “Before increasing a product’s price, we run simulations to understand whether customers would switch to other brands within the portfolio, switch to a competitor brand, change the buying pack sizes or reduce purchase volume,” Sachin said.
However, achieving this level of AI sophistication was not always possible. Before Databricks, each business unit used different platforms and technologies, resulting in significant inefficiencies. Teams developed models in silos, often using local environments such as Anaconda, with no standardized platform or way to monitor models at scale. The growing volume of data caused challenges during model training and validation. “When we wanted to scale an application, that required several worker nodes. We found it challenging to run the entire end-to-end pipeline in one go,” Sachin explained. Security and compliance were concerns, particularly when dealing with open source libraries. Mondelēz turned to the Databricks Data Intelligence Platform to centralize and modernize their data and AI foundation — setting the stage for enterprisewide transformation.
A unified platform for scalable, secure, AI-powered innovation
The Databricks Platform offered Mondelēz the flexibility to scale their workflows efficiently without the usual infrastructure headaches. Sachin said, “With Databricks, the resources we need just spin up and provide what’s needed on demand.” The machine learning engineering team is empowering better collaboration and experimentation; enabling security, governance and reusability; powering generative AI at scale; and simplifying infrastructure with Google Cloud Platform (GCP).
Mondelēz uses Databricks Notebooks to drive cross-functional collaboration among data scientists, engineers and analysts. Model experimentation and training are managed through MLflow, with thousands of models tracked using MLflow Model Registry. With this, Mondelēz is managing up to 20,000 models in their environment — with a complete understanding of which are production-grade and which aren’t. The snacks company also uses AI Playground to compare outputs across different large language models (LLM) — such as Gemini, Llama and Mistral — enabling side-by-side evaluations of generative AI outputs. Meanwhile, Databricks Assistant, a context-aware AI that allows users to query data using a conversational interface, assists engineers and data scientists with troubleshooting. “Databricks Assistant has been useful in resolving coding issues because it helps us see what’s missing in our code and how to adapt it. You get a faster response, so there is a time-saving benefit.”
Data security and model governance are foundational to Mondelēz’s AI adoption. With Unity Catalog, the manufacturer enforces fine-grained access control across their cloud environment — particularly critical as they begin scaling their generative AI use cases. Databricks also eased the compliance burden with pre-certified libraries for model development. “It reduces the risk that comes with open source. We don’t have to worry about installing everything ourselves,” Sachin said.
Mondelēz is taking full advantage of Mosaic AI, Databricks’ agentic framework for developing GenAI applications. One major project in development is “SnackGPT,” a domain-specific assistant for brand and category teams to ask natural language questions like “What were our top-selling products last year?” or “Why did sales drop in Q1?” The assistant extracts information from syndicated point-of-sales data and other internal sources, using Gemini Pro models for summarization and reasoning. Early pilots have shown promising results in answering user queries in real time and have accelerated insight generation for Mondelēz’s category and brand managers.
To ensure efficiency and consistency on platform usage, Mondelēz is integrating directly into the native Databricks services — for smoother LLM access to context-rich embeddings. They are also adopting the Mosaic AI Gateway to manage usage throughput and establish guardrails for safe, compliant GenAI deployment. Mondelēz runs Databricks on Google Cloud, which enhances operational efficiency through tools like Lakehouse Federation. According to Sachin, “It has given us an ability to see the entire ecosystem without needing to create a new connection for each product. Now, we just reuse existing integrations, and it’s much more efficient.”
Reduced technical debt and improved experimentation on 20K models
Today, teams across sales execution, supply chain and revenue management are building and deploying ML models faster, more collaboratively and with stronger governance. With Databricks at the center of their transformation, Mondelēz has significantly reduced operational inefficiencies, improved scalability and laid the foundation for trusted AI and ML at a global scale. One of the clearest signs of progress is the scale of experimentation now possible. “We are registering close to 20,000 models, and around 3,000 of those are in production,” Sachin shared. “Now, we can centrally see which models are in production, which are experimental and which are not production-grade. It gives us the scale we need to move fast and stay in control.”
The centralization of workloads on the Databricks Platform has also improved data access and governance across projects. “With Unity Catalog and Lakehouse Federation, we now have a centralized pane of access that lets us connect to and govern data access across multiple projects. The technical debt of managing all these systems has been reduced,” Sachin added. Beyond operational efficiencies, the company is also using analytics to demonstrate the business value of machine learning in real terms — like an uplift in sales from product recommendations or improved forecasting accuracy in the supply chain. “If a business was able to sell one additional product because of a model recommendation, we can directly measure that uplift,” Sachin said. “It’s a revenue growth story.”
Mondelēz has no plans to slow down, with two key priorities underway. They are planning to implement Delta Sharing to make reusable segmentation models and workflows available across their regional teams, reducing duplication of effort. They’re also preparing to implement Lakehouse Monitoring to track latency, accuracy and hallucinations from GenAI responses — all via a preconfigured dashboard tailored to their needs. Other innovations are already on the roadmap, including content generation through images for product packaging and LLM-powered promotion planning tailored to specific geographies.
With a unified data intelligence platform in place and a clear vision for innovation, Mondelēz is poised to continue leading the industry into its AI-driven future. Sachin concluded, “Databricks has been so easy for the team to operate, empowering us to meet the needs of the business.”