Unlocking critical healthcare for high-needs seniors
Decrease in voluntary disenrollment for members targeted for earlier primary care physician reassignment
Reduction in voluntary disenrollment during annual enrollment
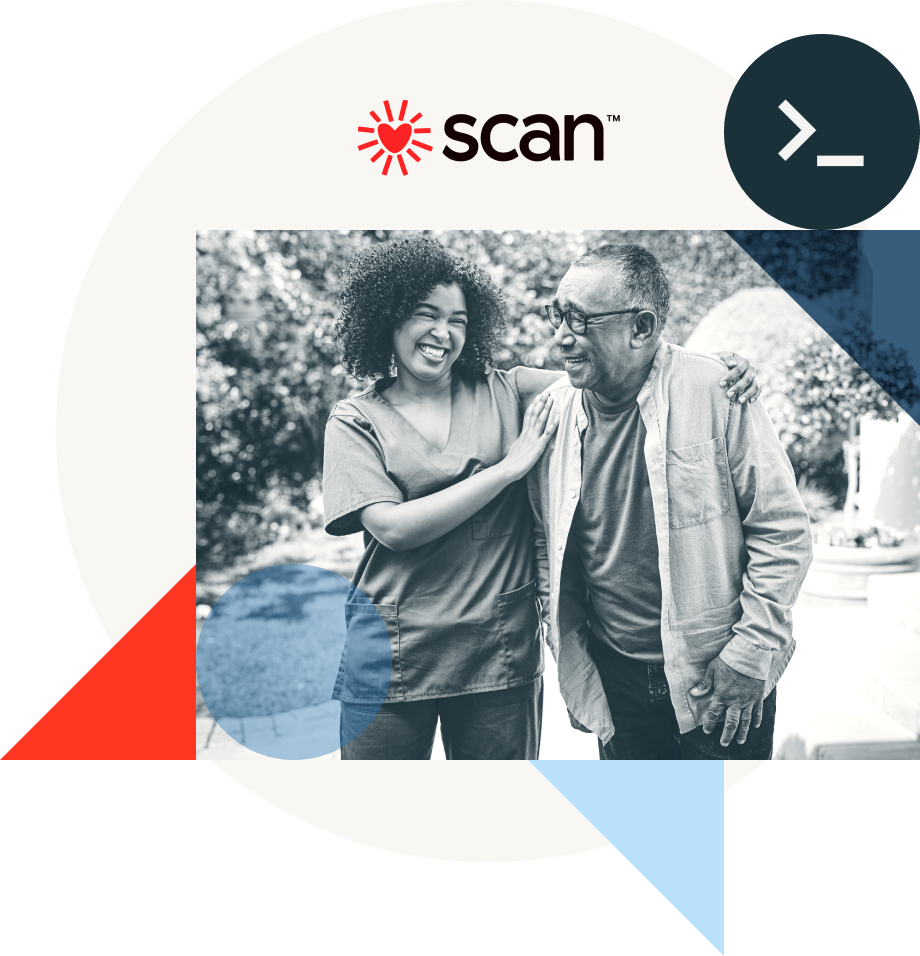
SCAN Health Plan is one of the nation’s largest not-for-profit Medicare Advantage health plans. Founded by a group of senior activists who were dissatisfied with their lack of access to healthcare services, SCAN Health Plan (SCAN), known then as the Senior Care Action Network, has always been fueled by their mission to keep seniors healthy and independent.
SCAN began to build out their data science efforts to help unify unstructured and structured data from sources including electronic medical records (EMR), claims submissions and member engagement data. The vision? Paint a complete picture of every member under care — all while maintaining the member focus of the organization, including affordability, access and wellness.
SCAN looked to Databricks to quickly extract valuable data insights to facilitate preventive healthcare. Additionally, data-driven internal decision-making helped lower operational costs without impacting the quality of member care.
Experiencing the barriers of unstructured data and silos
Healthcare is navigating technological advances, changes in healthcare policies, demographic shifts, and cost and efficiency concerns. SCAN Health Plan (SCAN) wanted to ensure that empowerment remained at the forefront of their customer experience by engaging members in their own care decisions, preparing them to handle health and life changes, and fostering open and productive communication channels. However, all of this became increasingly difficult to facilitate as the organization continued to scale their offerings and grow their member base, which came along with an ever-growing mix of structured, unstructured and semi-structured data from multiple systems of record.
Despite it being a current obstacle, SCAN’s unstructured data (e.g., call center notes, medical charts, PDF medical documents) could ultimately serve as the major unlock for the organization as it expands into the clinical realm. One of the reasons why the not-for-profit Medicare Advantage plan was already developing a natural language processing (NLP) pipeline throughout the various peaks of the COVID-19 pandemic — and continues to do so — was to uncover member behavioral patterns and detect recurring themes in member service interactions. Unfortunately, the NLP model couldn’t reach the next level of development until data access and the pipeline were improved first. As Sajid Chaudhary, IT Director of Analytics Technology and Architecture at SCAN, explained, “We didn’t have the tools or the infrastructure to analyze the unstructured data we were dealing with, which sat around 20 to 25% of our total data — and who could say what value it could uncover?”
SCAN set about building their Insights Platform, a home for their NLP, machine learning (ML) and further artificial intelligence (AI) investments. The Health Care Informatics team began SCAN’s work in data science. Led by Shan Rui, Director of Research and Analysis at SCAN, the team uses ML models to predict members’ major health events and self-management of conditions. Since the company was reaching a roadblock with their unstructured and siloed data, SCAN’s models were unable to home in on the specific issues members were facing, hindering the company from providing the experience they aspired to deliver. Whether it was a member’s desire to change primary care physicians or transition to palliative care, the inability to analyze for and anticipate these events was disruptive to SCAN’s mission to keep seniors healthy and independent. But by implementing an easy-to-maintain lakehouse architecture with the Databricks Data Intelligence Platform, patterns in member behavior could be easily identified. This gave SCAN’s Analytics Technology and Architecture team time to evaluate new data sources, track model pipeline runs and improve data governance to fuel the Insights Platform.
Gaining data visibility with lakehouse architecture
As healthcare became increasingly intertwined with data, SCAN felt their organization was hitting a wall with conventional analytics, which served more as an “odometer,” according to the team — telling them how fast they were going rather than helping them find valuable insights to improve experiences, prioritize preventive health and strengthen decision-making. Plus, as the company continued on their trajectory of growth, the teams were increasingly looking to streamline operations and administrative tasks while remaining cost-efficient.
With the Databricks Platform, a combination of the best elements of data lakes and data warehouses, the company was now positioned for a quick turnaround on their AI initiatives in SCAN’s Insights Platform. Built on an open source lakehouse framework, the Databricks Platform eliminated data silos among SCAN’s data teams, ensuring everyone had access to and visibility into the same data to maintain infrastructure and foster collaboration.
For example, because Databricks SQL applies data warehousing techniques to data lakes, SCAN is able to unify their data within a single system, using Delta Lake to build reliable and performant data pipelines at scale. Then, SCAN can leverage Databricks Unity Catalog with Collibra, a data governance platform, to further augment data visibility, sharing and quality with enhanced security and governance measures. Users of varying technical backgrounds can search Collibra to find authoritative definitions and reports that could answer their questions. Finally, integrations with Tableau and Power BI enable the visualization and reporting of said data to measure the impact of changes made to existing processes.
Now, SCAN can continue to develop model pipelines on their Insights Platform. This lets the company discover the issues impacting members at scale, predict for voluntary disenrollment or chronic condition exacerbation, and advance the member experience and clinical use cases. Moreover, their model pipeline continues to serve high-needs seniors — triggering case management, home care and other responses when necessary. According to Chaudhary, “Databricks allows our technology team — Analytics Technology and Architecture — to speak in the same language as the Customer Experience and Insights team and the Healthcare Informatics team. These three groups can work together to deliver the right data to meet the needs of our modeling efforts.”
Validating members throughout their medical journeys
With Databricks, SCAN has the resources they need to build and maintain a successful lakehouse architecture, with the end goal of uncovering data patterns to enhance the member experience. For their teams, the Databricks Data Intelligence Platform has reduced the cost of IT operations while simultaneously increasing their ability to scale SCAN’s offerings in a meaningful way. As Corrine Stroum, Director of Consumer Experience and Insights at SCAN, puts it, “You can’t always prevent it just because you predict it — but if we can ease those experiences for our members, then the work is worth it. When these undesirable events happen, they know that we’re alongside them during the entire journey.”
SCAN sees Databricks as the great unifier, an anchor for all of their data science work in the Insights Platform. Yet, it (proudly) recognizes veering off the traditional path of the typical healthcare use cases. Stroum adds, “A lot of our models are created to provide great experiences for our members, not to push around paperwork. That’s what I like about working with Databricks. You’re on the cusp of everything that’s changing in the market, and I feel a sense of comfort in that stability that that next feature will simply show up in our Databricks Platform.”
The models Stroum refers to have had impactful results for member satisfaction and retention. For example, the NLP model helped SCAN learn that members who enroll online frequently call regarding their primary care physician assignments. With this information, SCAN called online-enrolled members early in their relationship to identify the best primary care provider for them, reducing the likelihood that new members will leave in the future by 3.6%.
Model pipelines also help SCAN identify members at risk for voluntary disenrollment, especially during the annual enrollment period in the fall season. With insights gleaned via NLP, SCAN can easily identify members dealing with undesirable experiences in obtaining care or in using their supplemental benefits and intervene with a call campaign. Using this model, the team decreased the disenrollment of high-risk members by 4.59%.
As they look to the future, SCAN sees themselves expanding their relationship with Databricks — already including MLflow and Delta Sharing with affiliated provider organizations in their roadmap for next year — and their use cases, as the not-for-profit continues to refine the member experience and expand their business lines.