Smarter Roads, Smarter Drivers
operational efficiency across the entire machine learning lifecycle
team productivity through cross-team collaboration
to days: faster time-to-market of new features
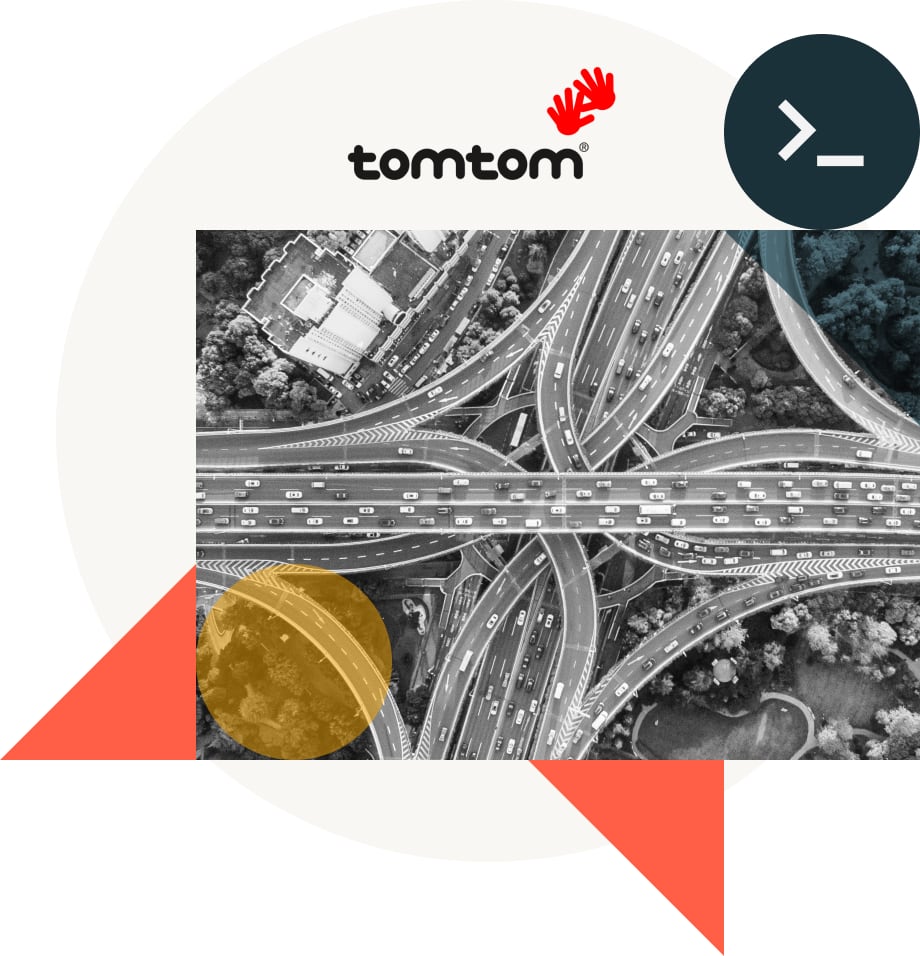
While data is unlocking new opportunities to improve the driving experience with navigation software and services, TomTom struggled to scale IT operations to support the demands of their data science teams. With Databricks, they have simplified infrastructure management while boosting data science productivity, allowing them to develop new products that help customers to safely get to their destinations faster.
Sensory data difficult to analyze at scale
TomTom’s mission is to create a world free of congestion and a better driving experience. In order to do that, they need to understand driving behavior from end users while optimizing the operational costs of their services. However, due to the large scale of their sensor data from vehicles, providing insights and performing advanced analytics was quite challenging.
- Massive volumes of data with over 600 million devices that generate over 80 billion geo-coordinates with timestamps and meta data collected every single day. This amounts to over 150 trillion data points.
- Struggled to scale operations to support data science efforts. As a result, time-to-insight was slower than they required to drive innovation — particularly for their autonomous driving use case.
- Data engineering spent too much time configuring clusters and maintaining infrastructure. This slowed data flow to the data science team for machine learning and analytics.
Databricks provides scalable platform for data and ML
TomTom uses the Databricks Data Intelligence Platform which has fostered a scalable and collaborative environment across data science and engineering, allowing data teams to more quickly innovate and deliver ML-powered innovations that feed into their navigation and automotive services and products.
- Fully managed platform with automated cluster management simplifies the infrastructure and operations at any scale.
- Collaborative notebook environment with support for multiple languages (SQL, Scala, Python, R) enables a diverse team of users to work together in their preferred language.
- Native support for MLflow enables data science teams to easily replicate experiments, track model performance, and rapidly iterate across their models in a systematic fashion.
- Native support for Delta Lake allows their data engineering team to deliver ACID transactionality to their data.
Helping develop smarter transportation solutions
With Databricks, TomTom has been able to harness the insights within their various streams of data to create innovative technologies that keep the world moving.
- Improved operational efficiency: Features such as auto-scaling clusters and support for Delta Lake and MLflow has improved operations from data ingest to managing the entire machine learning lifecycle.
- Better cross-team collaboration: Shared notebook environment with support for multiple languages has improved team productivity.
- Faster time-to-insight: Databricks has improved time-to-market, allowing them to accelerate prototype to production from weeks to days.