Webinar
Automating the Analysis of Digital Pathology Images with Deep Learning
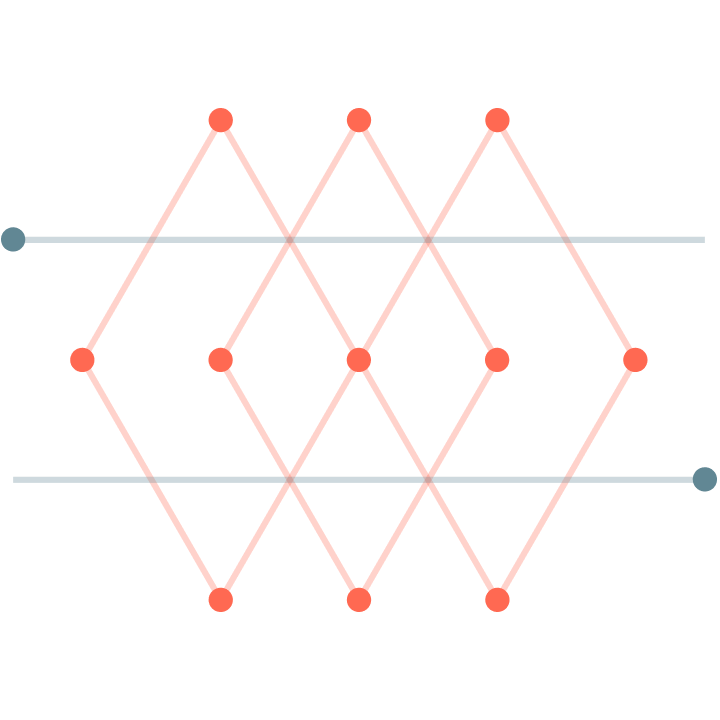
Available on demand
How to build an end-to-end deep learning pipeline for whole slide image analysis with Databricks Machine Learning Runtime and MLflow
Today, microscopic scans of tissue samples can be rapidly digitized at a low cost. These high-resolution images provide researchers and clinicians with rich information to help detect the presence of cancer, develop new therapeutics and more. However, most of this work requires labor-intensive human review of these images. Deep learning can augment these workflows by interpreting thousands of images in a matter of minutes.
Despite the promise of deep learning, healthcare and life sciences organizations struggle to implement automated digital pathology workflows for the following reasons:
- It’s slow and cost prohibitive to process large image files (e.g. 1–2 GB per slide)
- Deep learning pipelines are hard to parallelize and can takes weeks to train a model
- Tracking and reproducing experiments across research labs is a challenge
Fortunately, the Databricks Unified Data Analytics Platform along with popular open-source projects Apache SparkTM, Spark Deep Learning Pipelines and MLflow make it easy to build a scalable deep learning pipeline for medical image analysis.
Join this webinar to learn:
- How deep learning can be used to automate digital pathology image analysis
- How to use Databricks’s ML Runtime to process thousands of whole slide images in minutes
- How to train an image classifier to detect cancer metastases in tumor segments
- How MLflow can be used to easily track and reproduce clinical experiments
Speakers
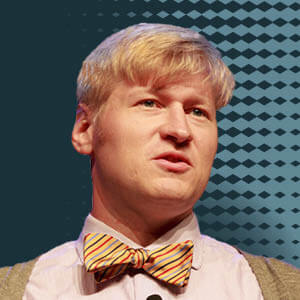
Frank Nothaft
Technical Director of Healthcare and Life Sciences
Databricks
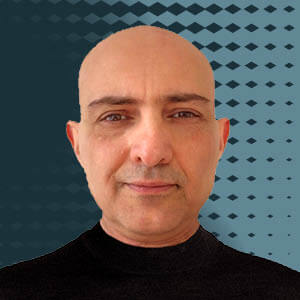
Amir Kermany
Healthcare and Life Sciences Solution Architect
Databricks
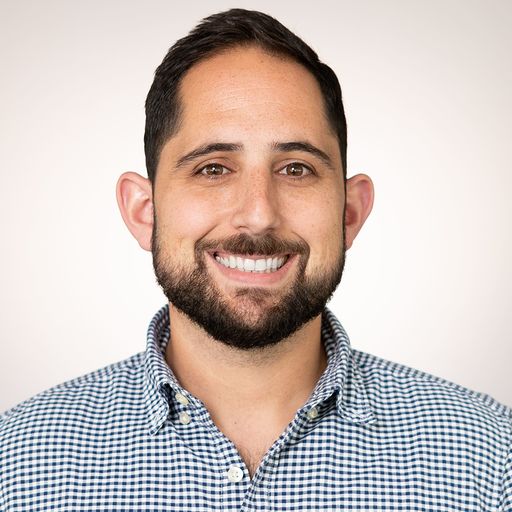
Michael Ortega
Industry and Solutions Marketing Lead
Databricks