Virtual Event + Live Q&A
Delta Lake: The Foundation of Your Lakehouse
Bring reliability, performance and security to your data lake
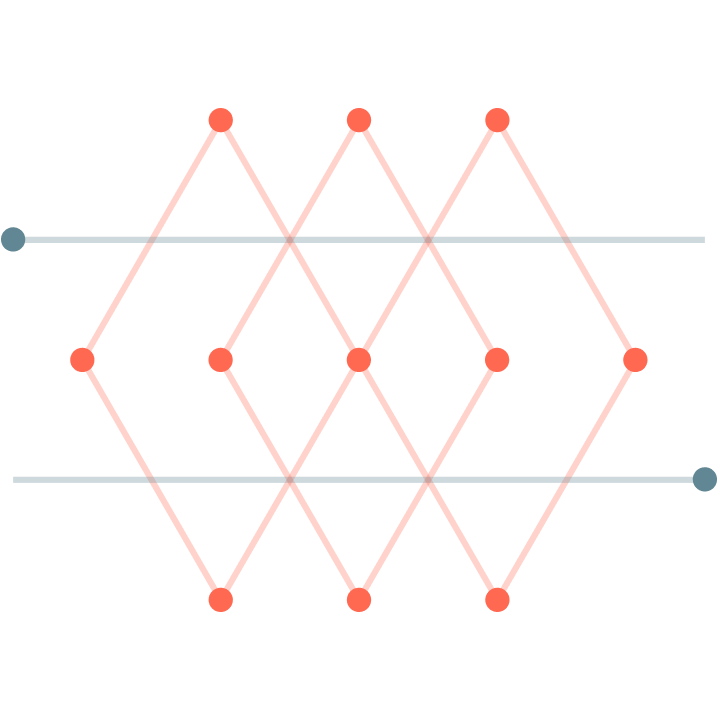
Available on-demand
As an open format storage layer, Delta Lake delivers reliability, security and performance to data lakes. Customers have seen 48x faster data processing, leading to 50% faster time to insight, after implementing Delta Lake.
Watch a live demo and learn how Delta Lake:
- Solves the challenges of traditional data lakes — giving you better data reliability, support for advanced analytics and lower total cost of ownership
- Provides the perfect foundation for a cost-effective, highly scalable lakehouse architecture
- Offers auditing and governance features to streamline GDPR compliance
- Has dramatically simplified data engineering for our customers
Speakers

Himanshu Raja
Product Management
DATABRICKS

Sam Steiny
Product Marketing
DATABRICKS
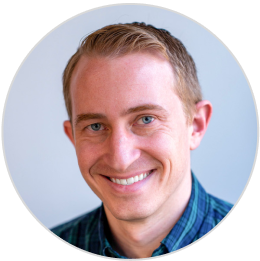
Brenner Heintz
Product Marketing
DATABRICKS

Barbara Eckman
Software Architect
COMCAST