Databricks for Industry
No-compromise data analytics and AI solutions purpose-built for your industry
Discover the lakehouse for your industry



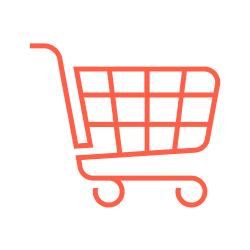
Browse all industries
Hide filters
🤔
No results available. Try adjusting the filters or start a new search.
reset the list
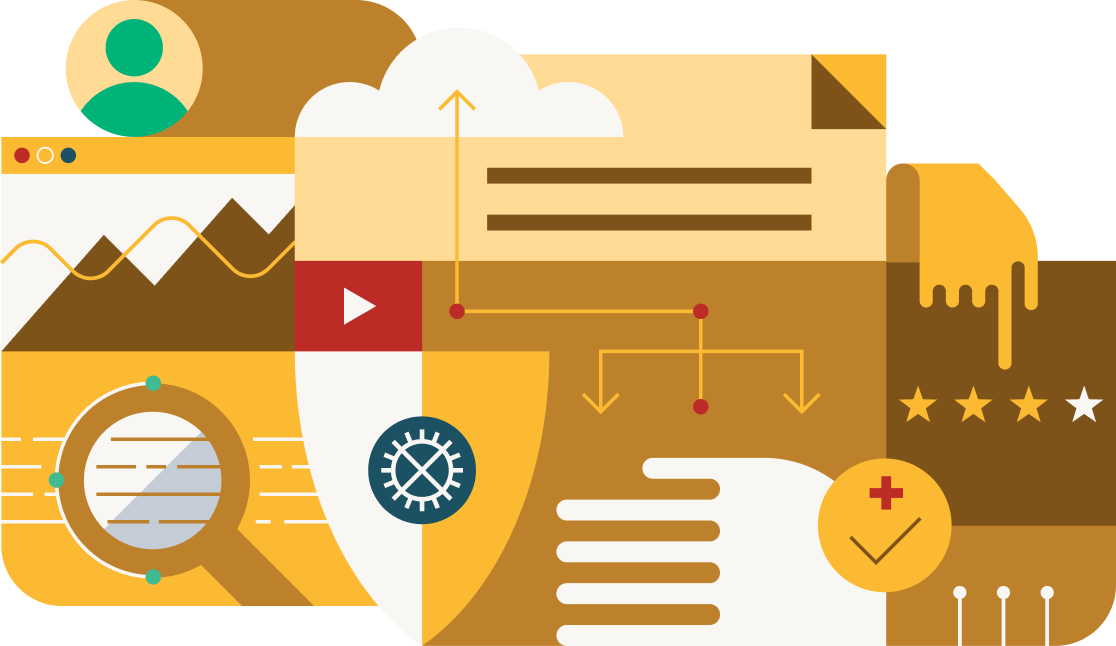
Industry Solutions
From idea to proof of concept in as little as two weeks
Databricks Solution Accelerators are purpose-built guides — fully functional notebooks and best practices — that speed up results. Databricks customers are saving hours of discovery, design, development and testing, with many going from idea to proof of concept (PoC) in as little as two weeks.
Ready to start?
Explore what Databricks can do for you by starting of with a free trial.