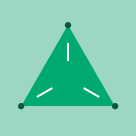
Jumbo Transforms How They Delight Customers With Data-Driven Personalized Experiences
This is a collaborative post between Databricks and Jumbo. We thank Wendell Kuling, Manager Data Science and Analytics at Jumbo Supermarkten, for his contribution.
At Jumbo, a leading supermarket chain in the Netherlands and Belgium with more than 100 years of history, we pride ourselves on a ‘customers first, data second’ approach to business. However, this doesn’t mean that data isn’t central to our mission. Rather, our organization and data teams are completely built around customer satisfaction and loyalty. Supermarkets operate in an extremely competitive and complex space, in which all components of the customer experience require optimization, including inventory, assortment selection, pricing strategies, and product importance per segment.
When we rolled out our loyalty program, the amount of new customer data points that started coming in got our data teams to rethink about how we optimize the customer experience at scale. At its core, Jumbo seeks to delight customers and deliver optimal grocery shopping experiences. Running differentiated store formats on one hand, and personalizing messages and offers to customers on the other, made it impossible to continue working in the traditional way. That’s where data analytics and AI come in: they help us to make decisions at the scale required for personalization and differentiation.
With the rollout of our revamped customer loyalty program, we were suddenly able to better understand a wide range of our individual customer preferences, such as which products are most important and which are often forgotten, as well as the best time of day to communicate with customers and on which channel. However, as data volumes grew exponentially, our analytics and ML capabilities began to slow as we weren’t equipped to handle such scale. Increased data volumes meant increased complexity and resources required to try to handle it from an infrastructure perspective, which impacted our ability to deliver insights in a timely manner. Long processing and querying times were unacceptable. After years on a traditional statistical software package connecting to a traditional RDBMS and analytics in Jupyter notebooks, we knew that if we wanted to best use this data and deliver shopping experiences that make a difference, it was time for us to take steps to modernize our approach and the underlying technologies that enable it. We needed a platform that was able to crunch through customer-level data and train models at a scale much more than we could handle on our individual machines.
In addition to needing to modernize our infrastructure to help us thrive with big data analytics, we also needed better ways to increase the speed from concept to production, decrease onboarding time for new people, collaborate and deliver self-service access to data insights for our analysts and business users to help serve insights around pricing, inventory, merchandising, and customer preferences. After looking into a variety of options, we selected the Databricks Data Intelligence Platform as the right solution for our needs.
From foundational customer loyalty to exceptional customer experiences
With Databricks Data Intelligence Platform implemented, we now run a substantial number of data science and data engineering initiatives in parallel to turn our millions of customers into even more loyal fans.
As an example of data products exposed directly to customers, we’re now able to combine, on a customer level, information about purchases made online and offline which was very challenging before. This omnichannel view allows us to create a more comprehensive recommendation engine online, which has seen tremendous engagement. Now, based on past purchasing history as well as first-party data collected with consent, we can serve product-relevant recommendations that pique the interests of the consumer. Obviously, this is great from a business perspective, but the real benefit is how happy it’s made our customers. Now, they’re less likely to forget important items or purchase more than they need. This balance has significantly increased customer loyalty.
One example of data products that have helped improve the customer experience, we continually run an algorithm that proactively suggests assortment optimizations to assortment managers. This algorithm needs to run at scale at acceptable costs, as it optimizes using large quantities of data on physical store and online customers, the overall market, financials and store-level geographical characteristics. Once opportunities are identified, it is presented in combination with the same breadth and depth of data upon which it was based.
From a technical perspective, the Databricks lakehouse architecture is able to drive these improved experiences with the help of Microsoft Azure Synapse. Together, this combo has allowed us to manage, explore and prepare data for analysis for automated (proposed) decision-making, and make that analysis easy to digest via BI tools such as Power BI. With deeper insights, we have helped spread a more meaningful understanding of customer behavior and empowered our data teams to more effectively predict the products and services they would want.
Databricks is now fully integrated into our end-to-end workflow. The process starts with a unified lakehouse architecture, which leverages Delta Lake to standardize access to all relevant data sources (both historical and real-time). For example, Delta Lake also helps to build data pipelines that enable scalable, real-time analytics to reduce in-store stockouts for customers and at the same time reduce unnecessary food waste due to over-ordering perishables, such as fresh produce, that won’t sell. At the same time, Databricks SQL provides our data analysts with the ability to easily query our data to better understand customer service issues, processed with NLP in the background, and relate these issues to the operational performance of different departments involved. This helps us to make improvements faster and improve the customer experience.
We would not have been able to accelerate our modernization efforts without the expert training and technical guidance from the Databricks Academy and Customer Success Engineer, which acts as a direct injection of knowledge for our data science department. This deeper understanding of how to leverage all our data has led to significant improvements in how we manage our assortment, supply chain, make strategic decisions, and better support our customers’ evolving needs.
Excellence has no ceiling when driven by data and AI
By focusing on improving the customer experience through Delta Lake, we were enabled beyond our initial expectations. The steps we’ve taken to modernize our approach to how we can use data have really set us up as we continue to transform our business in a way that pushes our industry forward.
It’s remarkable to see how quickly data and AI capabilities are becoming the new normal and we are now well-positioned to realize the direct impact of our efforts to be data-driven. The output of sophisticated machine learning models is considered ‘common practice’ within 4 weeks after introduction. And the speed of idea to production is counted in weeks nowadays, not months.
Going forward, we’ll continue to see the adoption level increase, not just at Jumbo, but across all of commerce. And for those who are also embarking on a data transformation, I would strongly recommend that they take a closer look at improvement possibilities in the experience they're providing customers. Feeding back your analytics data products into operational processes at scale is key to transforming all areas of the business forward and successfully into the future.