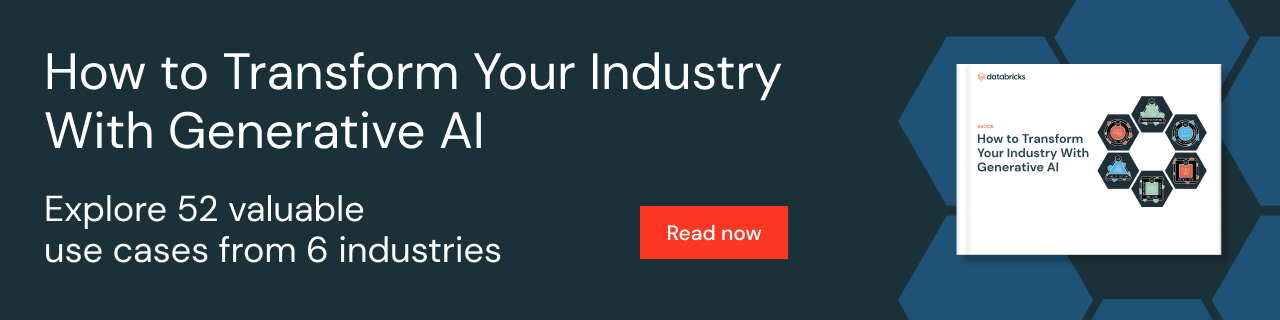
What is generative AI?
Large Language Models (LLMs) such as ChatGPT have garnered a ton of attention lately along with the broader topic of Generative AI. Their ability to generate seemingly thoughtful, well-informed and even human-like responses to user-provided prompts is truly amazing. Many organizations recognize these as a game-changing technology and organizations like OpenAI (who crafted ChatGPT) are using that buzz to drive revenue.
To understand this new wave of AI, let's start by defining it. Generative AI as these technologies have come to be known is capable of producing new and seemingly original creative works be it written text, images or music. These AIs aren't just repeating what's been done already. Instead, these models are trained on large volumes of examples and from these learn the basic patterns behind how humans craft such content, arriving at never before seen or observed constructs that we would have previously understood as an expression of human creativity. But unlike the simpler AI of the past that could determine which word follows the next or apply a stylized filter to an image, these AI have the capacity to understand the broader context of the body of work being produced so that the content formed is far more coherent and cogent to the human interpreter.
ChatGPT has become the fastest-growing app in the world by recording 100 million users in two months since its initial launch and ushering in a new era in search. But ChatGPT is just one of many LLMs, and we're seeing companies like Microsoft and Google (Bard) quickly jump on the bandwagon of generative AI. We even publicized our own experiment with Dolly, an inexpensive-to-build LLM that exhibits a surprising degree of the instruction-following capabilities exhibited by ChatGPT, which we recently released to the open source community.
Is Generative AI Ready for Prime Time?
Generative AI is extremely promising and companies should be piloting it. It has the ability to retrieve general knowledge, develop a point of view, analyze certain types of information and more. But generative models have a few weaknesses we need to resolve before they're viable in commercial uses.
- They sound authoritative, even when they're not accurate. If you ask an LLM to provide contrasting opinions on the same topic, it will do so and sound confident in both. While LLMs can generate coherent text, they still struggle with understanding and generating text that accounts for broader contextual understanding. LLMs can only generate responses based on the information they have been trained on, which can result in limited knowledge or understanding on topics outside of their training data.
- Intellectual property rights. In many cases, LLMs are trained on text data that is owned by individuals or organizations who have legal rights to that data. These data owners may have proprietary or copyright interests in the data and may require that their rights be respected when the data is used to train large language models. And ownership of content that is generated by generative AI models is uncertain. The US Supreme Court recently ruled that AI generated images do not have IP protections.
- Cost and Energy Consumption: One of the biggest limitations of LLMs has been the cost (primarily computing) to train the models. Millions of dollars for each training. Not only is this economically infeasible to many firms, at scale it will create a major negative impact on the economy. (This is one of the primary reasons we built Dolly!)
- They can reinforce biases. LLMs have been known to reproduce biases present in the training data. This can lead to biased language generation or decision-making based on biased inputs.
Future Applications of Generative AI in Retail
Despite these challenges, we predict a strong future for generative AI and are working with customers today on a range of pilots.
What do we predict will be popular uses for generative AI? The list is as endless as the ChatGPT knowledge base, but here are some key categories we're hearing or expect retailers to invest in generative AI for:
- Personalized marketing: Every customer is unique. Generative AI can be used to improve the customer experience and provide an individualized message to consumers in email or online with products that are relevant to the shopper.
- Virtual fitting room. Generative AI can be used to generate custom images that match a shoppers interest with available products. Shoppers can have generative models render available products from the catalog on a picture of themselves.
- Chatbots/Automating customer service. Transcripts from call center interactions, product information, and real-time order information can be used to tune LLMs to reduce the load on call center agents.
- Scheduling customer delivery and installation. Order a new home theater and need it delivered and installed? LLMs can be integrated with mobile applications, web sites or even in-store kiosks to make it easier for retailers to coordinate delivery and installation options with customers. They can access configuration requirements, need parts, resource requirements and more to ensure delivery and installation is successfully completed in one visit.
- Catching security threats and account takeover attempts. Generative AI can be used to analyze customer messages or other communications for signs of fraudulent activity, such as phishing attempts or social engineering.
- In store assistant. As anyone who has visited a home improvement store can attest, asking "what aisle is X product in," often gets the wrong answer. LLMs can be integrated with web and mobile applications and leverage data on store layouts, mixed with current inventory information, to more accurately respond to these requests.
- Inventory and demand planning: As one of the biggest pain points for retailers, there is massive potential to optimize demand prediction and inventory planning with generative AI - helping retailers avoid overstocking or understocking. Generative AI can analyze sales data and make recommendations for what levels of inventory should be stocked at which stories and help retailers forecast trends by analyzing historical data, consumer sentiment and competitive data.
- Product development: In order to create unique and innovative products that stand out in a saturated market, retailers can leverage Generative AI to create new product designs based on customer preferences, market trends, and other data. We expect to see document reviews play a big role here, leveraging product reviews, document discovery, contract reviews, etc, to drive prodcut roadmap and development.
- Generating actionable insights. Retailers no longer have to depend on an employee's ability to interpret a report. LLMs can be trained to interpret information in structured form, analyze it, and return relevant insights in plain language. And by connecting an LLM with a predictive model, it can begin to automate the analysis of inventory positions, labor staffing, customer engagement or any number of additional situations.
- Code development. Generative AI can be used to develop code! Developers can work with generative models to develop a base code that they enhance, greatly improving their productivity.
These are just a start. As LLMs incorporate techniques such as langchain, they can be used as a user friendly way to invoke far more complex AI models. An LLM can trigger a customer churn model that generates and returns an analysis, and then provide a written interpretation of the data from that model. Overall, generative AI has the potential to help retailers improve customer engagement, increase sales, and optimize operations, making it a valuable tool for the industry.
How does Databricks fit in to generative AI?
The intention of Dolly was to show the approachability of generative AI — that anyone can take a open source large language model (LLM) and give it ChatGPT-like instruction following capabilities using high-quality training data.
With Dolly, we were able to prove that a freely available, open source LLM that's been around for a couple of years could be trained up on a relatively small (and certainly accessible) volume of data on a fairly small Databricks cluster. The point of this demonstration was to show that organizations have a choice in how they approach this technology and that they don't need to be beholden to outside parties in order to obtain this functionality.
We're in the earliest days of the democratization of AI for the enterprise, and much work remains to be done, but we believe the technology underlying Dolly represents an exciting new opportunity for retailers that want to cheaply build their own instruction-following models and leverage generative AI in their business.
How can you prepare to take advantage of this new technology?
LLMs and Generative AI technologies have the potential to revolutionize the way businesses operate and create value. But there is still a ways to go. What is critical for retailers is to prepare their business for this new wave of technology now. Retailers can do that by:
- Developing an AI strategy - Having a clear AI strategy that aligns with your overall business will be critical. Retailers should outline how AI can be used to enhance customer experience, improve supply chain and inventory management, and drive product innovation and explore new use cases for automation and decision making.
- Investing in data infrastructure - Any future use of generative AI will require massive amounts of data to train models to operate effectively. Retailers should invest in building a data lakehouse that can collect, store, and manage all types of data (structured, semi-structured, unstructured) and supports the BI and AI use cases this technology will leverage.
- Capture data in your Lakehouse. Generative AI models are generalized. What will make them powerful and relevant is data specific to companies. Companies should be investing in capturing and annotating data in anticipation of these generative models.
- Pilot. While generative AI may not be ready for commercial use today, they are sufficiently good to support experimentation. Piloting these tools now will ensure you are ready to adopt them when they're mature.
What to learn more? Visit our site to learn about the Lakehouse for Retail or learn how you can harness LLMs yourself in our webinar: Build Your Own Larch Language Model Like Dolly.