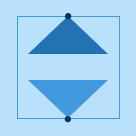
Personalization provides organizations a means to more directly connect with customers' needs and preferences. In a crowded retail landscape, such targeted engagement is often seen as a key differentiator, attracting customers, elevating their spend, and increasing their loyalty to particular brands. Consumers themselves increasingly express a desire to be seen and heard by the outlets with which they spend their time and money, and even when pulling back spend in the face of economic concerns, 52% of consumers in a recent survey stated they expect a better experience from brands in return for their spend.
This shift towards personalization has even extended into the realm of consumer packaged goods. With relationships to consumers mediated by retail outlets, CPG manufacturers have long pursued mass-marketing strategies intended to increase demand for their goods across more general populations of consumers. But recent shifts in consumer habits and brand loyalties and the growth of private label brands have created a sense of urgency amongst manufacturers to connect more directly with consumers. The rise in retail media networks and consumer interest in DTC models all create an opportunity for this kind of relationship, but with these opportunities comes a new expectation for personalized engagement.
The Need for a Customer Data Platform (CDP)
The key to delivering personalized experiences is access to timely, high-quality data about the consumer. Information such as a customer's transaction history is critical but so are data on returns, product feedback, browsing history, customer service records, and so much more. These data, internally generated as well as externally captured, provide a 360-degree view of the customer from which new insights and experiences may be derived.
While developing such a Customer-360 platform sounds straightforward, the reality is that it is not. Customer identity is often an elusive concept, large data volumes and complex data structures may make accessing information challenging, and sophisticated insights such as behavioral segment assignments and personalized product recommendations derived from base data in the 360-degree view may require access to sophisticated analytic capabilities. Many organizations with ambitions to establish a customer data platform (CDP) have found their efforts falling short, with only 14% of organizations achieving what they would qualify as success according to a recent survey.
But there have been some successes, and as this space matures, we are seeing the emergence of a repeatable pattern that some refer to as a hybrid-CDP and others refer to as a composable-CDP.
With this pattern (which we will refer to as a composable-CDP), many core challenges such as customer identity resolution, the acquisition and integration of frequently employed data and the derivation of standard metrics are largely addressed by more-or-less off-the-shelf CDP technologies. High-volume and complex data are accumulated on more flexible Data Lake or Lakehouse platforms where data may be pre-processed for downstream integration into the core CDP system. Advanced analytics needs may be tackled by pushing a subset of the harmonized information from the core CDP back into the Lakehouse where models may be trained and predictive insights generated which are themselves pushed back into the core CDP environment.
While the composable-CDP entails quite a bit of data movement, it balances the strengths of the dual platforms, providing organizations the right combination of capabilities required to meet their Customer-360 needs.
Integrating Amperity and Databricks to Form a Composable-CDP
To illustrate the combined power of the composable-CDP architecture, let's examine a simple integration between Amperity, a market leader in the CDP space with a deep specialization in ML-driven customer identity resolution, and Databricks, the platform that defined the Lakehouse approach to analytic data management (Figure 1).
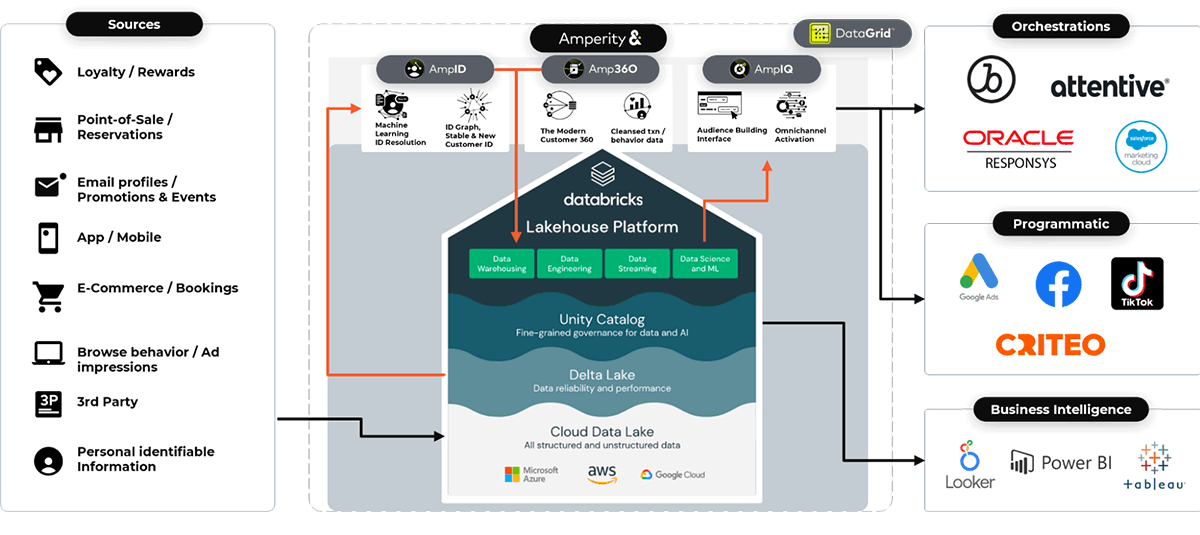
Leveraging Amperity's Databricks Delta table destination feature, data can easily be pushed and pulled between the Amperity CDP and the Databricks platform. We might leverage such an integration to push customer transactional history, enhanced by Amperity for reliable customer identification, to the Databricks environment. There, data may be used to train a model to generate customer-specific product recommendations. Those recommendations may then be pushed back to the Amperity CDP to be integrated into the Customer-360 where it can then be activated in a variety of channels like email, sms, push notifications, and paid media/advertising like Google, TikTok, Facebook, Instagram, LiveRamp, Criteo and more.
The key to this workflow is not simply the integration of the two platforms, but what each contributes to the end product, i.e. personalized product recommendations. While Databricks has long been recognized for its ability to train large and complex models such as those used in recommendation systems, the output of these models is only as good as the data they are trained upon.
Amperity's ML-based identity resolution capabilities are critical in ensuring that data associated with a customer are accurately attributed to them. A study of Amperity customers' legacy identity resolution strategies found that on average organizations struggle to correctly identify 23% of their customers representing 53% of the revenue associated with those brands. It's hard to extract the benefits of personalization when you can't properly align the data from your best customers (Figure 2).
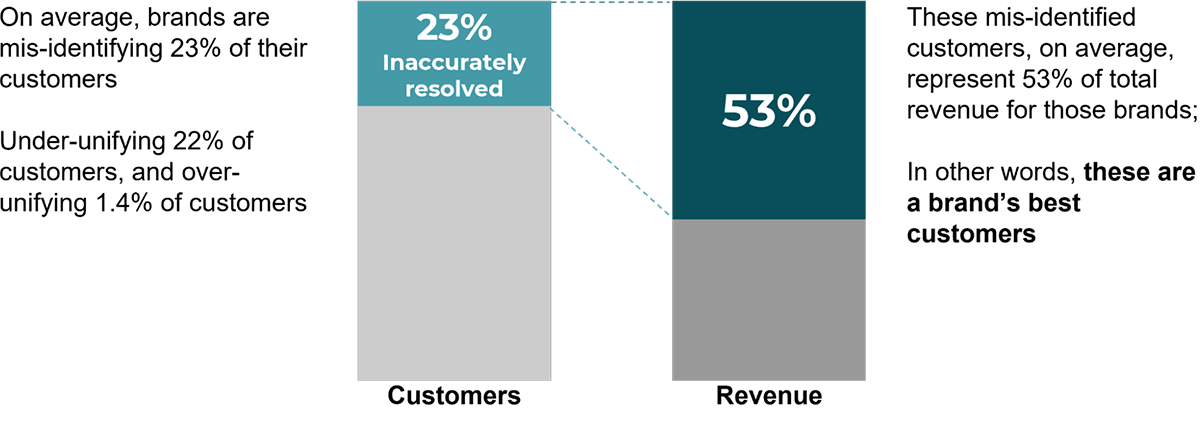
Want to See This in Action?
To demonstrate the power of combining the Amperity CDP with the Databricks Lakehouse, we've put together a demonstration notebook exploring the generation of personalized product recommendations in a composable-CDP architecture. In this notebook, we demonstrate exactly how data is pushed from the Amperity CDP to Databricks, how enhanced transaction history from Amperity is used to train a recommender and how recommendations generated in Databricks are pushed back into the Amperity CDP.
This is but one of many scenarios where our mutual customers are finding success in meeting their needs for a 360-view of their customers and creating a foundation for success in their personalization efforts. If you'd like to explore how Amperity and Databricks can help your organization achieve similar success, please contact either your Amperity or Databricks representatives.