eBook
Data Management in Financial Services
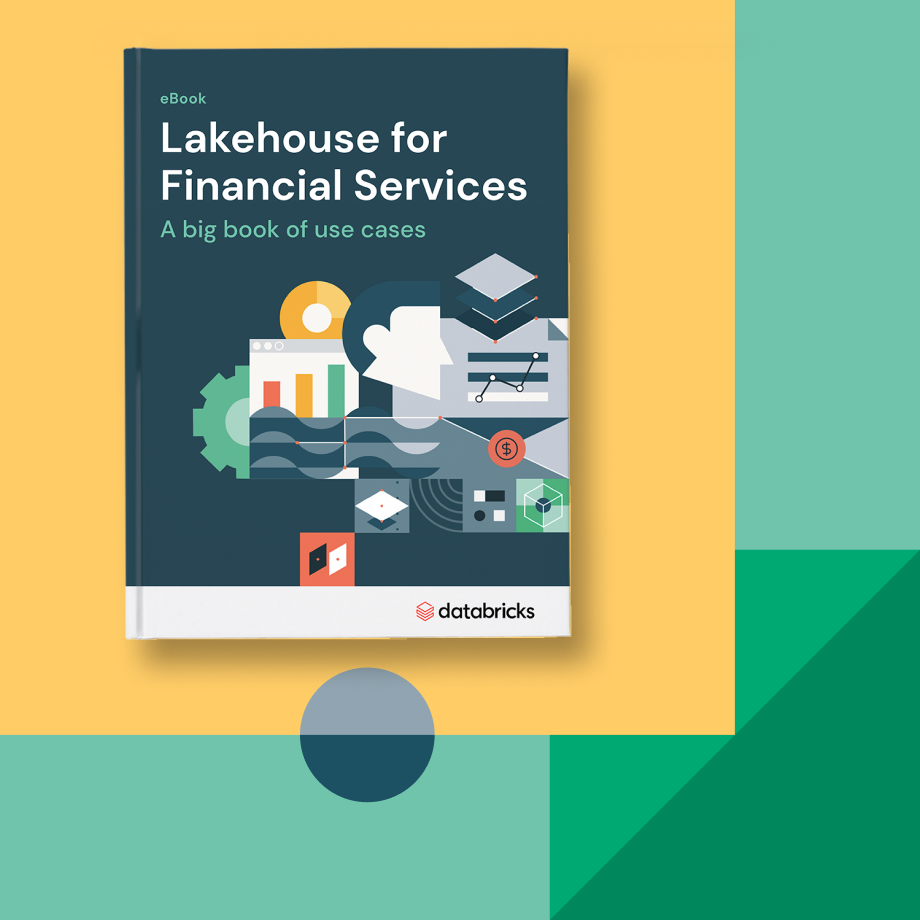
Big data is key to digital transformation. But in order to harness its full potential, organizations need to create specialized strategies for data management in financial services.
By adopting AI-driven data lakehouse platforms and applying data management best practices, your financial team can streamline processes and gain insights that accelerate success.
What is financial data management?
Financial data management is the strategic use of processes, policies and technology to effectively manage financial information.
With the assistance of specialized software and algorithms, organizations can consolidate their financial data and resources, maintain regulatory and legal compliance, and utilize business intelligence (BI) to generate accurate, insightful reports.
Master data management (MDM) refers specifically to the creation and maintenance of master data (i.e., customer data, asset data and partner data). Master data management for financial services focuses on cleaning, validating and centralizing master data, essentially creating an authoritative source of truth that exists as a single point of reference.
Why is data management important in financial services?
As we accelerate toward digitization, data is becoming ever more omnipresent and increasingly valuable for financial services.
Everything from building a modern risk management framework to cash flow management and forecasting fundamentally depends on 360-degree data visibility and rigorous data accuracy.
Without a centralized strategy for data management in financial services, you risk reducing the quality — and, in turn, the value — of your data. You also put your organization in danger of noncompliance, data breaches and data loss. All of which have the potential to devastate even the most thriving business.
Still not convinced? Let’s explore the importance of financial services data management in more detail.
Understanding the gravity of data management in the finance industry
Data management in financial services is maturing at scale, responding to the critical need for heightened security, stricter compliance and innovative transformation.
It resolves many of the challenges that financial institutions face in the digital-first climate, all while unlocking fruitful new opportunities that give businesses a competitive advantage. Let’s take a look at the specifics.
It guarantees conclusive internal and external reports
Your internal reports utilize an abundance of data to communicate everything from employee performance and operational efficiency to budgeting requirements and social media engagement.
Your external reports are just as data-reliant because they depend on data transparency and reliability for the accurate communication of regulatory compliance and company health.
With your organization’s profitability, reputation and all manner of executive decisions inherently reliant on data reporting, it’s critical that your internal and external reports have rock-solid validity. But without data management, how can you be sure that the data you present is accurate?
Data management strategies utilize various techniques, technologies and automations to centralize and normalize financial service data.
Financial data can be consolidated, cleaned, organized and distributed with streamlined centricity, hardened by strict data quality processes that guarantee data accuracy in every internal and external report.
It drives data-driven transformation of the business
Data scientists are often overwhelmed with data preparation. In fact, approximately 80% of a data scientist’s time is spent collecting, cleaning and organizing data in preparation for analysis. This leaves less time for more business-critical activities such as data mining and modeling.
Through the implementation of enterprise data management strategies and technologies, data scientists are able to spend less time completing tedious data preparation activities.
Instead, they can build data models and design patterns for real-time insights, refine algorithms to enhance data quality, and complete other time-critical tasks that drive real-world business transformations.
It allows the finance team to be independent and optimize resource management
It’s not uncommon for finance teams to lack data maturity, even in large, digital-first enterprises. And yet, the success of a financial team is heavily reliant on timely and accurate reporting, budgeting and analysis.
So why is it that finance teams still need to access data via other departments? Why do many finance teams still rely on outdated tools like spreadsheets to complete budgeting?
It’s largely due to poor data management implementations.
An effective data management strategy helps improve workflow efficiency. Centralization and consolidation (through the use of a scalable cloud lakehouse platform, for instance) eliminates data silos, minimizes IT reliance, and reduces on-premises, resource-intensive dependency.
Additionally, advanced lakehouse platforms provide an abundance of use cases for financial services, including risk management, hyper-personalization, ESG scoring and investment analytics.
With the power of data and analytics amalgamated into one platform, finance teams can improve daily workflows, solve strategic challenges, mitigate risks, and refine customer experiences with more efficiency than IT-dependent, resource-intensive teams.
It simplifies finance processes and builds standardized management
Ultimately, a robust data management strategy simplifies critical finance processes by aligning technology with the talent that powers it.
Process simplification is achieved not only through centralization and automation, but also through the newfound freedom that finance teams have to infuse finance processes with increased strategic value. With the power to define, secure and homogenize data, they can identify more opportunities and make better decisions.
Financial data management best practices
Successful data management strategies aren’t executed by accident. While every business strategizes its data management differently, there are some key steps and practices that are critical for long-term success:
Create a centralized data management road map
Your business’s data management road map should be a vision for your data management initiatives. It pinpoints the current state of your data handling practices, envisions your desired end goals and essentially details the steps that you need to take to get to where you want to be.
An effective data management road map looks a little like this:
Establish your objectives: What are your overall data management goals? What type of data will you require to meet these goals? What data analytics will you be running, and how will you measure the success of your initiatives? These questions are crucial for unifying teams and establishing clear steps for your road map.
Create robust data processes: Identify your data sources and establish the processes you’ll use for collecting, preparing, storing and distributing your data. You can utilize automated tools to streamline various data operations, such as data integration and redundancy. Make sure to define who will be using the data, how collaboration will be facilitated and how data will be presented to the end user.
Choose your technologies: Consider a specialized data management solution for financial services that combines data and AI into one consolidated platform. Ensure that it’s an open platform equipped to handle vast amounts of complex financial and alternative data. It will also need to support common financial use cases, such as ESG investing, secure data sharing and regulatory compliance.
Verify data governance: Strictly establish and communicate the rules, policies and procedures that govern data use in your organization. Pay close attention to data security, data privacy and data quality. The goal is to create a foundation of high-quality, accurate and consistent data, and then ensure that daily operations maintain this quality in alignment with legal and regulatory requirements.
Centralize and execute: Centralizing your data management road map involves more than just placing it in a central location. It entails clearly communicating your strategy across your business and onboarding non-IT teams to close knowledge gaps. Only then can you execute a data management strategy in which disparate teams can work collaboratively toward a shared end goal.
Ensure the data is available throughout the organization
Your data needs to be easily accessible across your entire financial services organization. But how do you achieve this?
Taking steps to reduce data silos is a good place to start. Siloed data occurs when data exists in multiple isolated locations, often on a departmental level. It can cause friction between teams, lead to inefficient processes and ultimately make your data less useful.
Centralizing and integrating your systems can be achieved with cloud data management solutions like the Databricks Lakehouse Platform. Lakehouse solutions are capable of pooling vast amounts of structured, unstructured and semi-structured data into one consolidated location.
Complete with user access controls and data classification methods, lakehouse providers ensure data accessibility while protecting sensitive data with strict adherence to data privacy and security practices.
Maximize data for accurate forecasting
Forecasts are at the heart of so many of our critical business decisions, influencing everything from hiring and budgeting to demand and sales. But accurate forecasting is critically dependent on the quality of the data that we have in our arsenal.
Irrelevant, redundant or invisible data poses considerable risks to the accuracy of forecasts. They decrease the reliability of analytics, resulting in poor business decision-making that can have far-reaching consequences.
A good data management strategy aims to improve data quality by verifying data accuracy, consistency and currency. In doing so, it maximizes the value of business data and lends itself to the formation of more accurate and informed business decisions.
Here are some examples of how you can maximize the value of your data:
Establish data quality standards across your organization:
Collaboratively define what constitutes “high-quality data,” identify data quality metrics and ensure that these are communicated across your organization.
Centralize master data to create a single source of truth: As part of your master data management strategy, you should aim to ensure that master data is easily accessible, understandable and accurate for end users. You can do this by centralizing records and creating online dashboards that update customer data in real time.
Determine a defined set of data values: When you have some employees writing “Mississippi,” others writing “MS” or “Miss”, and others misspelling the word entirely, it can lead to errors that seriously damage the quality of your data. Instead of relying on free-form inputs, create a defined list of values or options for common fields to maximize data consistency.
Foster a data-driven culture: Data-driven employees don’t just follow the rules associated with data quality; they have a clear understanding of why they’re so important. Provide training to ensure that everyone from your C-suite to your administrators understands how their role relates to data quality and security.
Invest in and bolster your finance team
While modern data management in financial services relies on AI-driven tools and technologies, it’s still your team of data experts that utilizes these tools to generate business-improving insights.
So investing in your finance team is an absolute must if you want to derive the most value from your data management initiatives.
By continually re-skilling and upskilling your current talent, you can nurture a strong, unified team of data experts. It’s also wise to identify any skills gaps in your organization and, if necessary, invest in fresh data science talent to close these gaps and fortify your data management capabilities.
Make backups of data in case of contingencies
Data loss is a persistent threat to financial services organizations and can have serious financial, reputational and legal repercussions. Not only that, but every lost data set and minute of business downtime is a potential missed opportunity.
Reduce the impact of data theft and data loss by implementing robust business continuity and disaster recovery plans. Backing up your data in multiple locations using cloud technology and online servers is a critical first step in protecting your business’s data against potentially devastating contingencies.
Lakehouse for Financial Services: Ascend and empower your business
Databricks Lakehouse for Financial Services is an open, cloud-based lakehouse architecture that’s built to simplify and centralize your data management.
It unifies data lakes and data warehouses into one central platform, eliminating data silos and streamlining data science, engineering, analytics, BI and machine learning.
With the ability to collect, process and transmit huge data volumes of any type, Databricks Lakehouse facilitates rapid data ingestion, drives stronger data governance procedures and ultimately guides transformative financial services innovations.