Data Analysis Workshop Series
Data Analysis with pandas
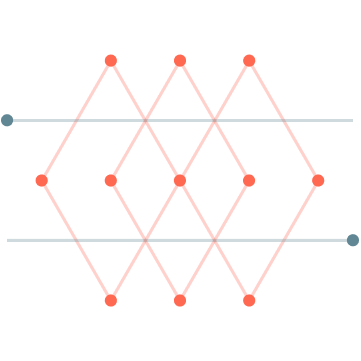
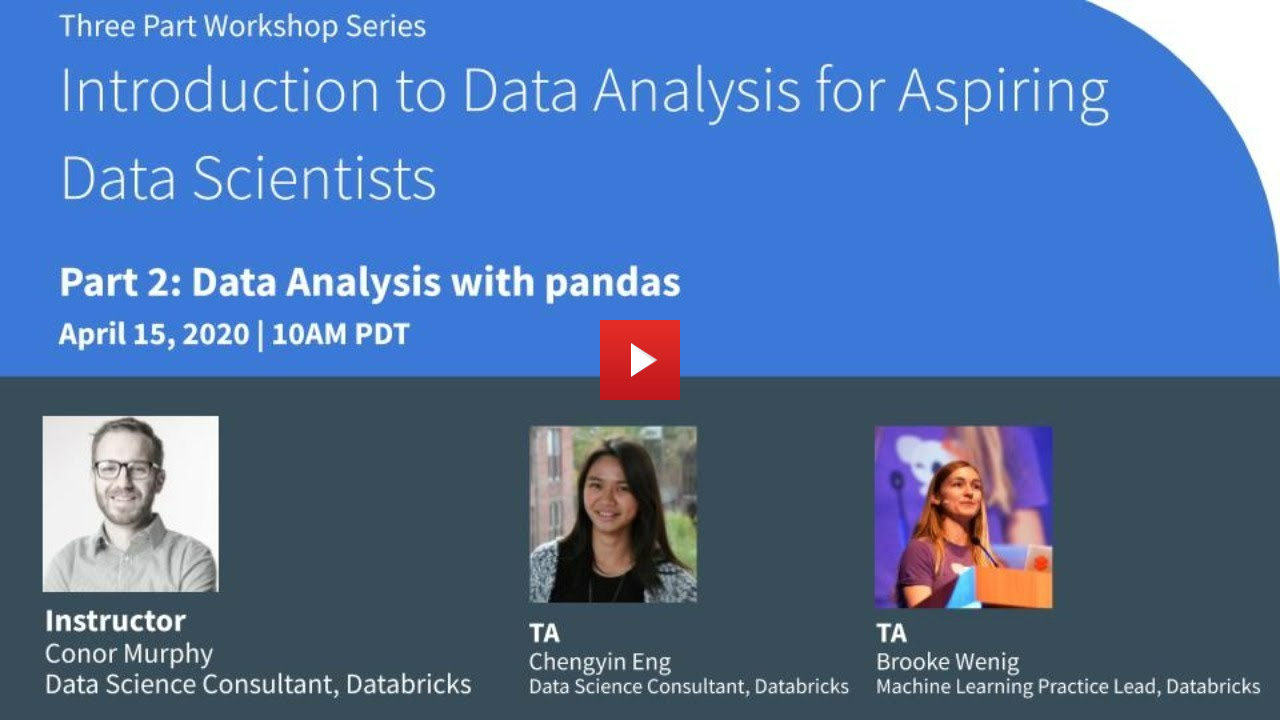
Workshop Details
This workshop is part two in our Introduction to Data Analysis for Aspiring Data Scientists Workshop Series.
This workshop is on pandas, a powerful open-source Python package for data analysis and manipulation. In this workshop, you will learn how to read data, compute summary statistics, check data distributions, conduct basic data cleaning and transformation, and plot simple visualizations. We will be using data released by the Johns Hopkins Center for Systems Science and Engineering (CSSE) Novel Coronavirus (COVID-19) (https://github.com/CSSEGISandData/COVID-19). Prior basic Python experience is recommended.
What you need: Sign up for Community Edition here and access the workshop presentation materials and sample notebooks here.
Although no prep work is required, we do recommend basic python knowledge. Watch Part One, Introduction to Python to learn about Python.
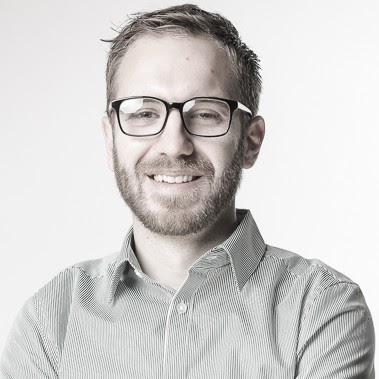
Instructor: Conor Murphy, Data Science Consultant at Databricks
Conor Murphy transitioned to the tech sector after spending four years leveraging data for more impactful humanitarian interventions in developing countries with a focus on business development. He managed a multi-million dollar portfolio of grants for The Rotary Foundation focusing on developing and analyzing impact measurements in economic development initiatives, evaluating program participation and translating academic research into institution policies.
He has held a variety of positions including a faculty role for Galvanize’s Data Science graduate program, principal data scientist and consultant for a number of startups and a data scientist and educator at Databricks. Outside of data, Conor is an avid skydiver who’s always looking for geeky ways to quantify his time in freefall.