Data Analysis Workshop Series
Introduction to Apache Spark
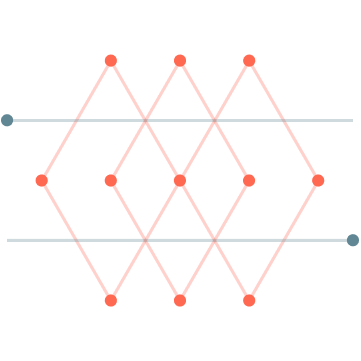
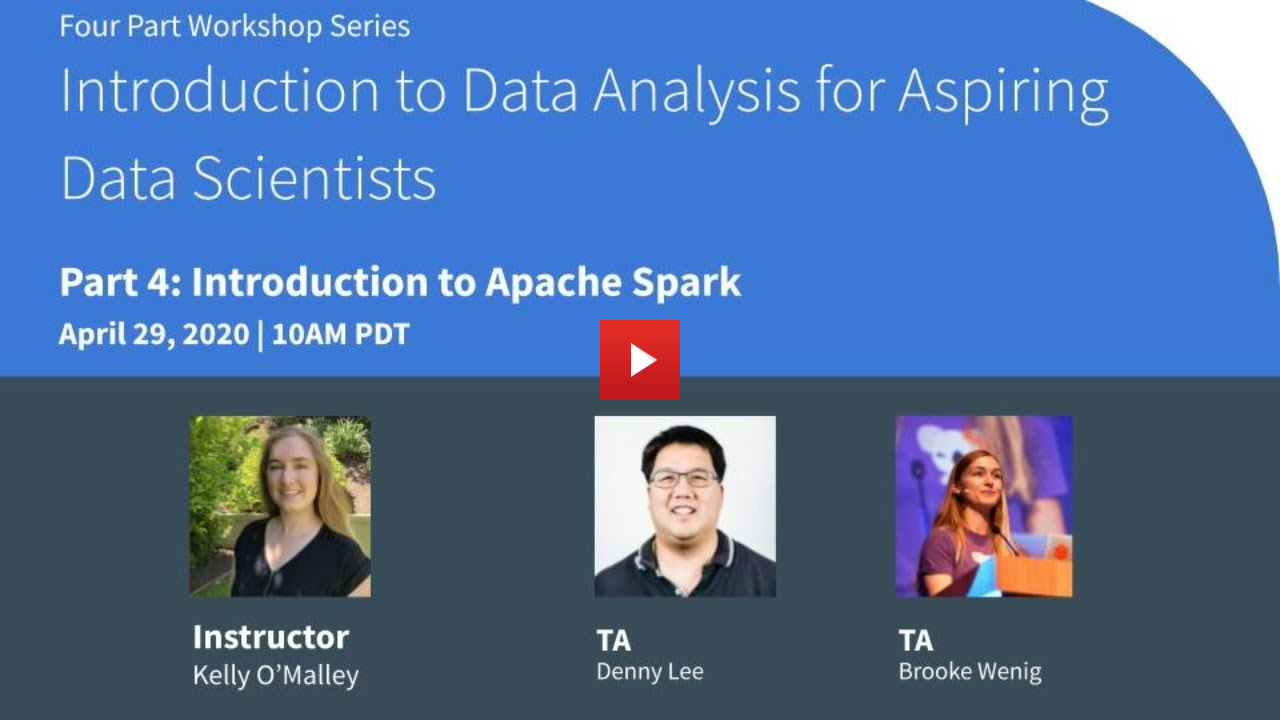
Workshop Details
This workshop is the final part in our Introduction to Data Analysis for Aspiring Data Scientists Workshop Series.
This workshop covers the fundamentals of Apache Spark, the most popular big data processing engine. In this workshop, you will learn how to ingest data with Spark, analyze the Spark UI, and gain a better understanding of distributed computing. We will be using data released by the NY Times (https://github.com/nytimes/covid-19-data). No prior knowledge of Spark is required, but Python experience is highly recommended.
What you need: Sign up for Community Edition here and access the workshop presentation materials and sample notebooks here.
Although no prep work is required, we do recommend basic python knowledge.Watch Part One, Introduction to Python to learn about Python.
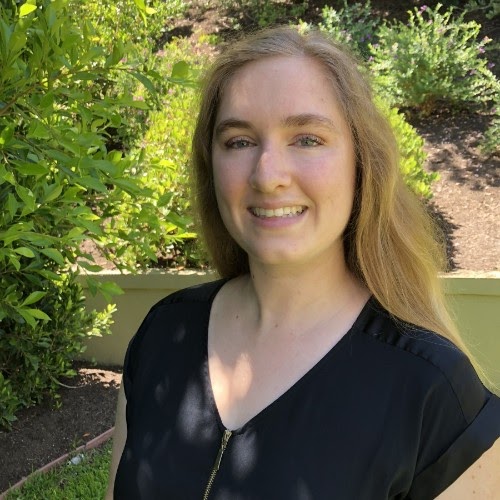
Instructor: Kelly O’Malley, Solutions Engineer at Databricks
Kelly O’Malley is a Solutions Engineer at Databricks where she helps startups architect and implement big data pipelines. Prior to joining Databricks she worked as a Software Engineer in the defense industry writing network code. She completed her BS in Computer Science at UCLA. Outside of the tech world, Kelly enjoys cooking, diy projects, and spending time at the beach.