Feature Store
The first feature store co-designed with a data platform and MLOps framework
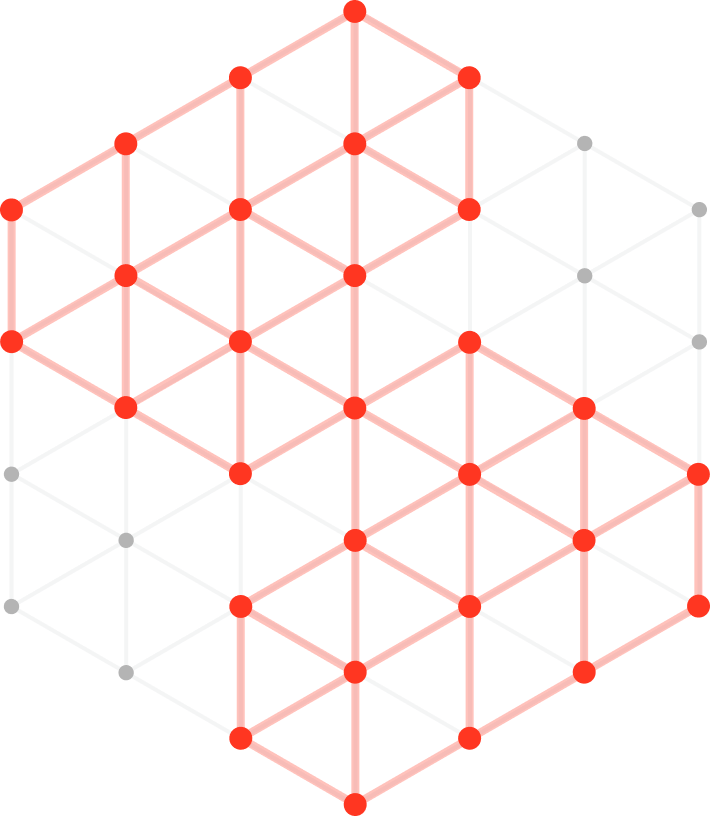
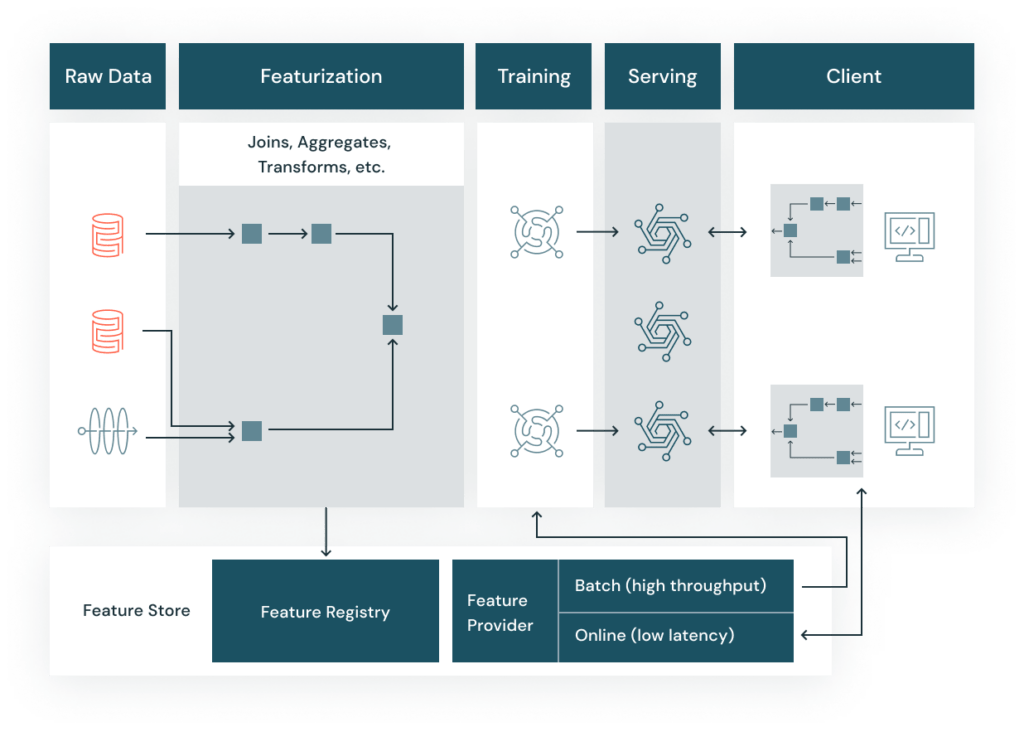
Provide data teams with the ability to create new features, explore and reuse existing ones, publish features to low-latency online stores, build training data sets and retrieve feature values for batch inference.
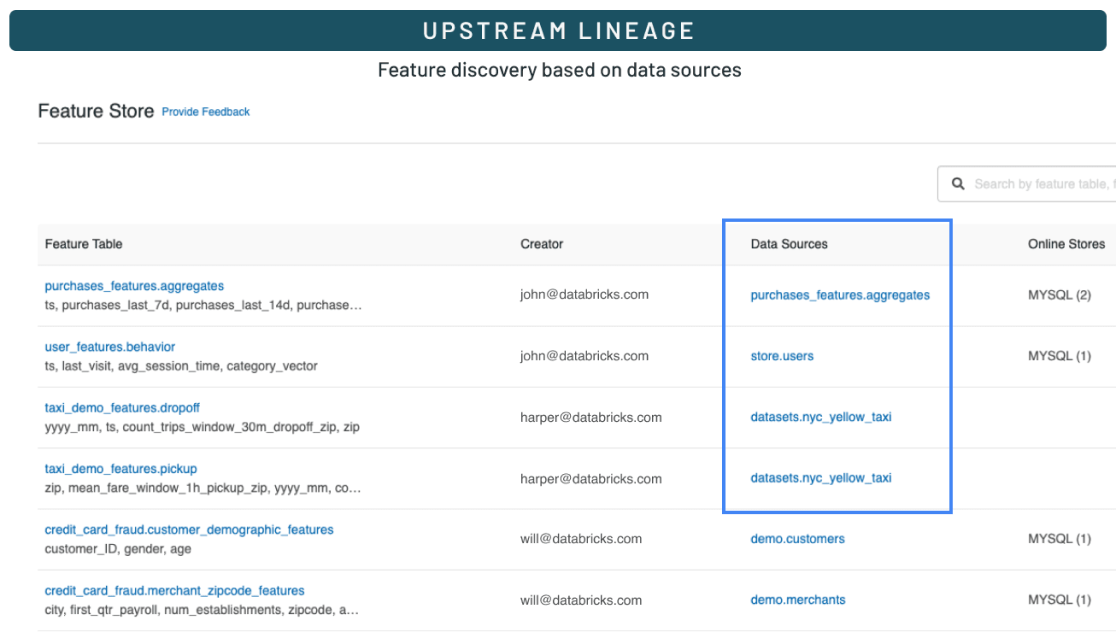
Features as reusable assets
Feature Registry provides a searchable record of all features, their associated definition, source data, and their consumers, eliminating considerable rework across teams. Data scientists, analysts and ML engineers can search for features based on the consumed raw data and either use features directly or fork existing features.
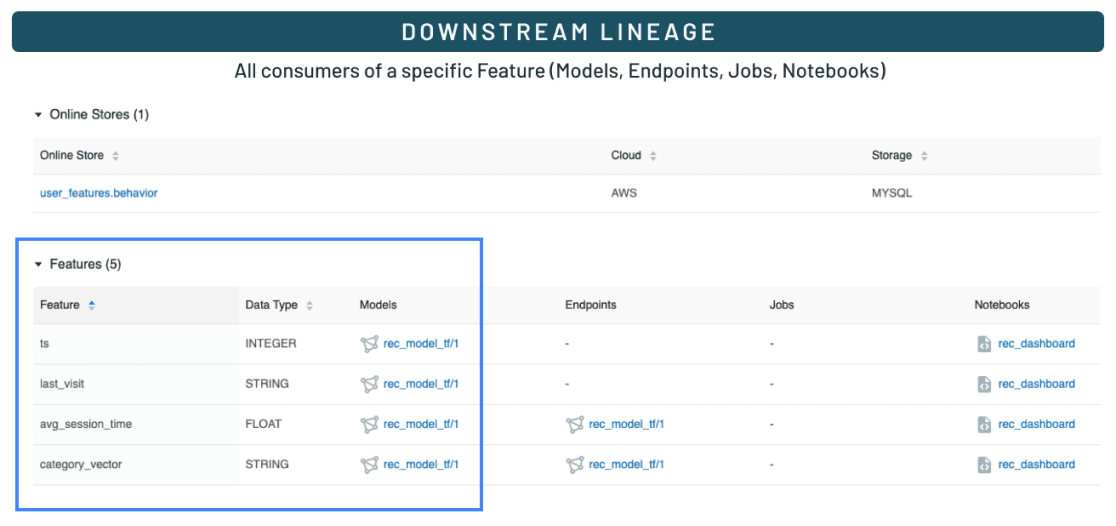
Consistent features for training and serving
Feature Provider serves the features in two modes. Batch mode provides features at high throughput for training ML models or batch inference. Online mode provides features at low latency for serving ML models or for the consumption of the same features in BI applications. Features used in model training are automatically tracked with the model and, during model inference, the model itself retrieves them directly from the feature store.
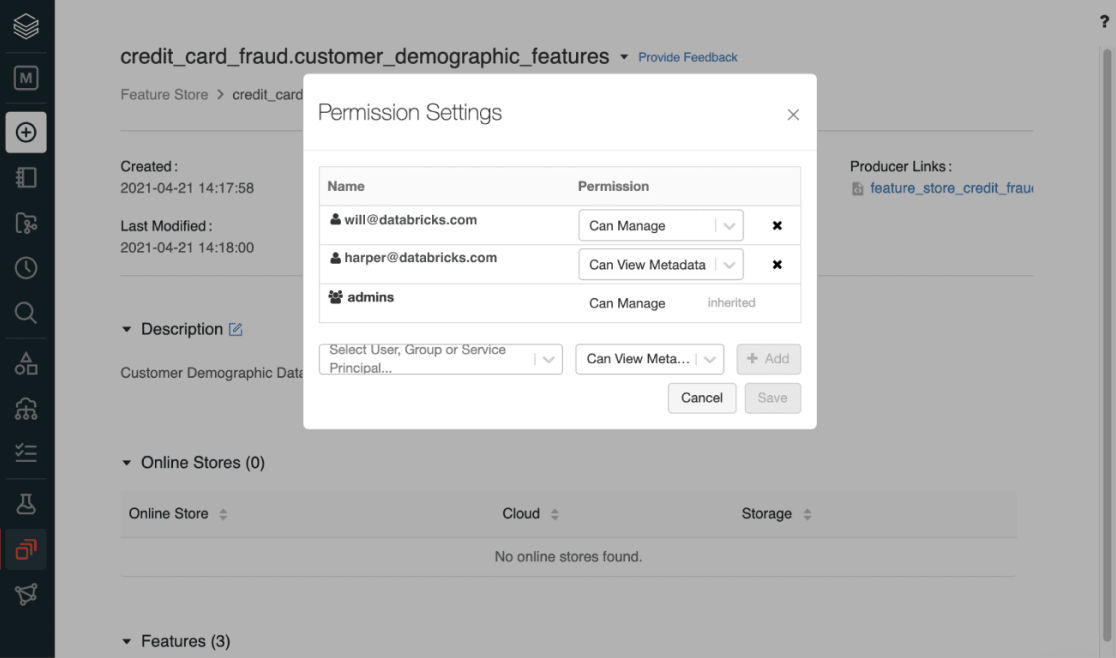
Secure features with built-in governance
Feature store integrations provide the full lineage of the data used to compute features. Features have associated ACLs to ensure the right level of security. Integration with MLflow ensures that the features are stored alongside the ML models, eliminating drift between training and serving time.
Resources
Virtual Event
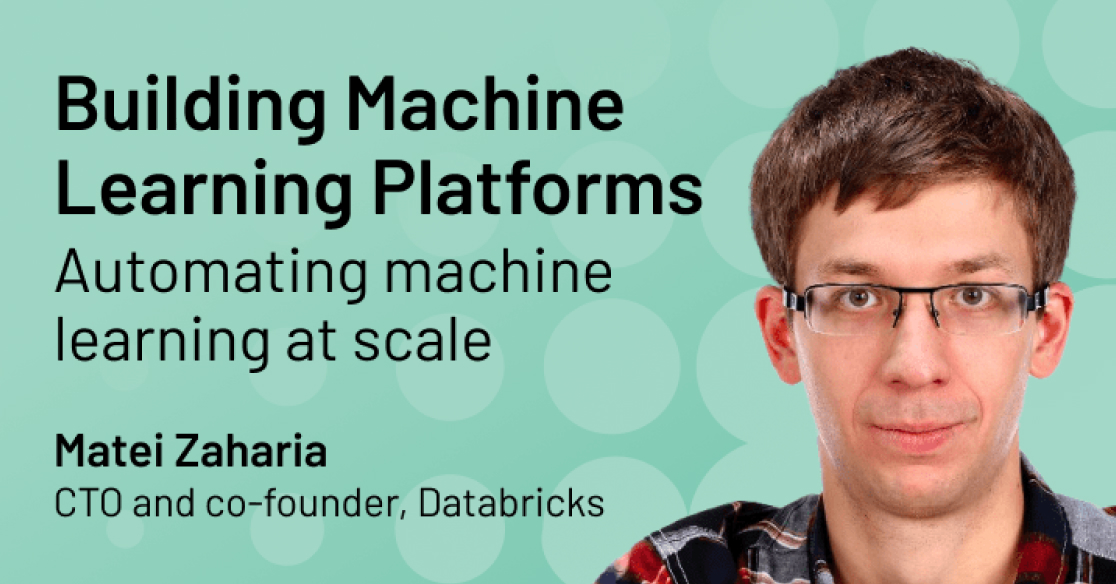
Documentation
eBooks
Ready to get started?